Disruptive businesses
Disruptive businesses
Artificial intelligence, the most human of intelligences
Artificial intelligence, the most human of intelligences
3 de febrero de 2023
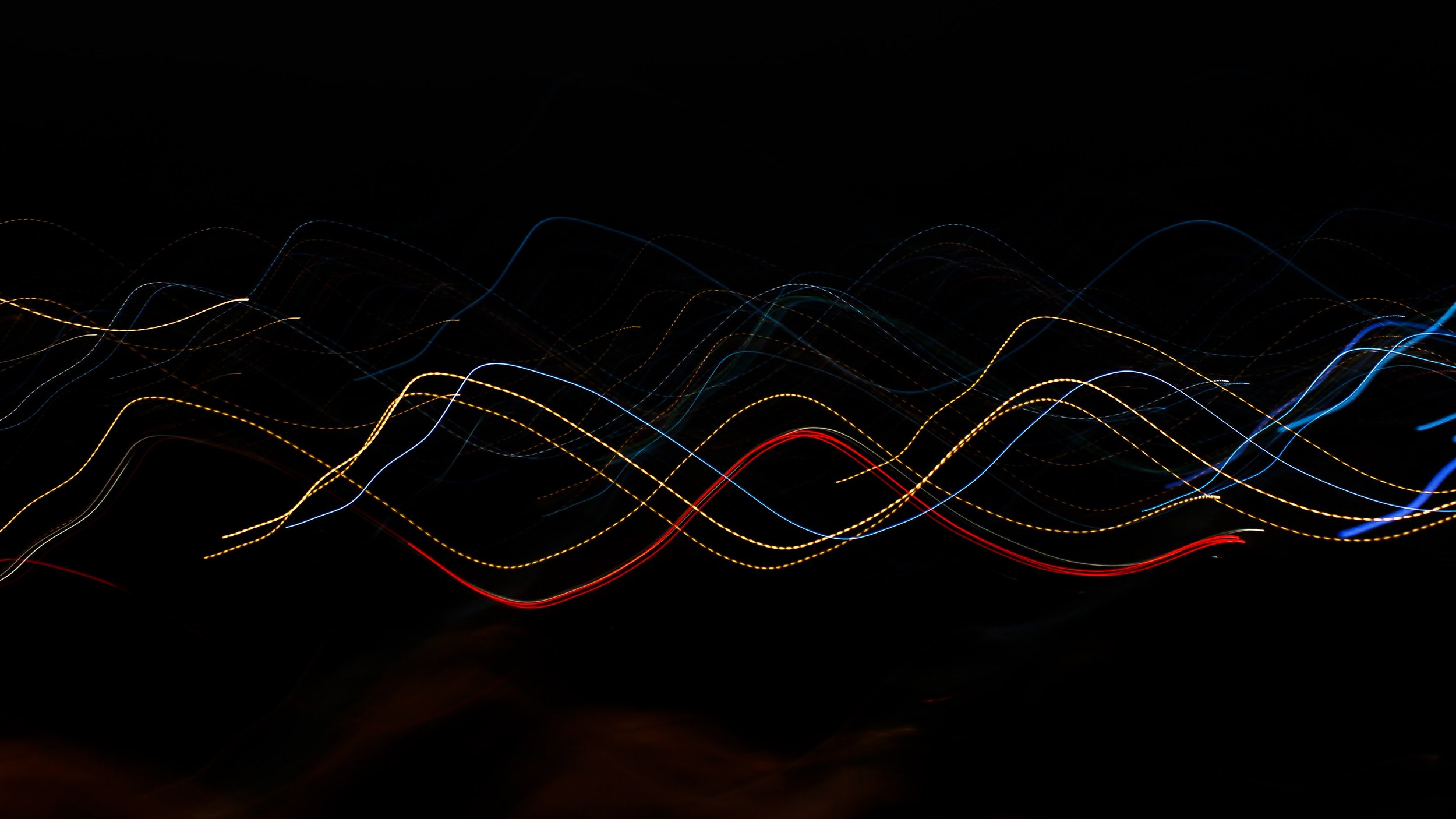
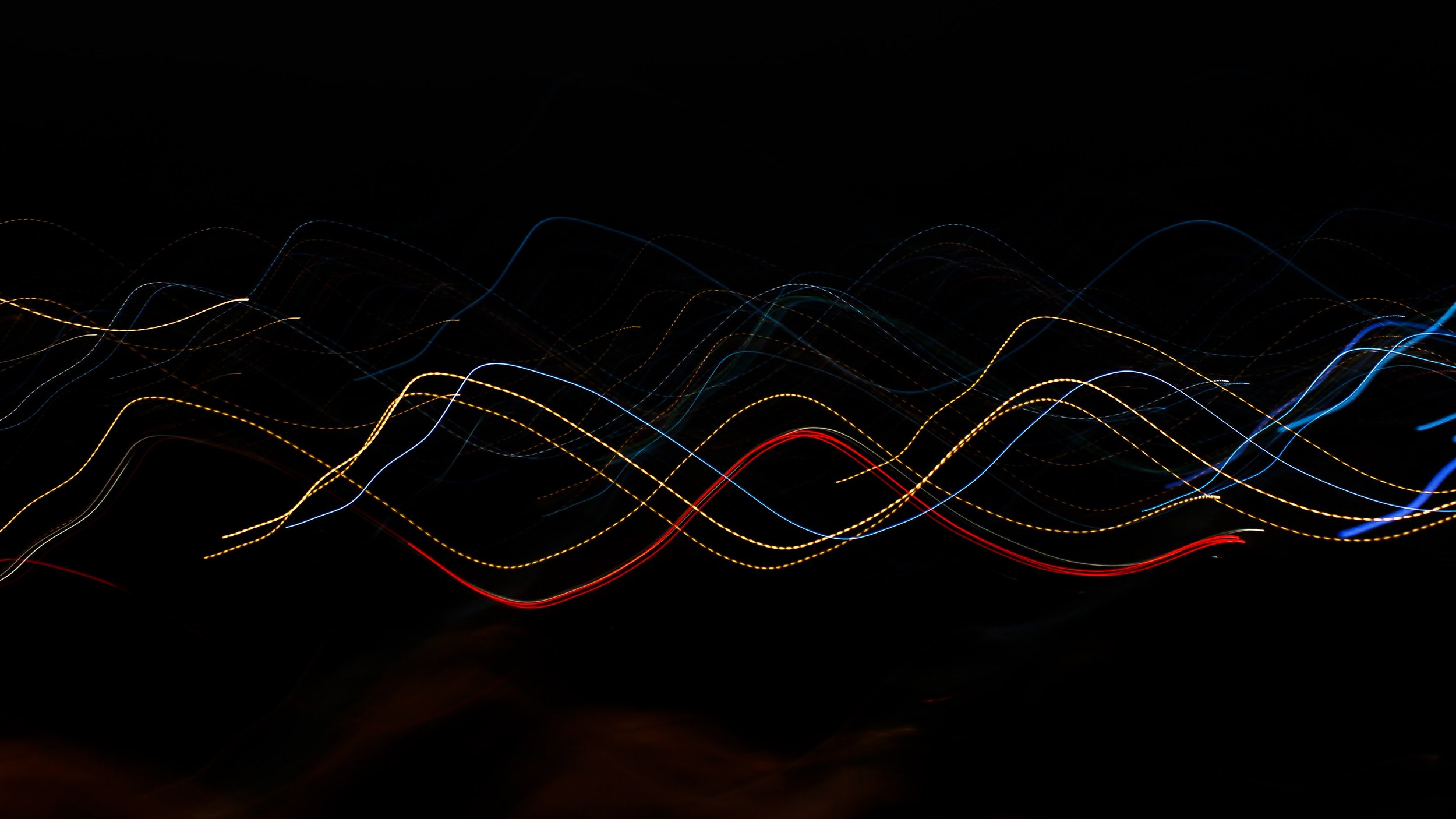
By Karina Gibert, director of the Intelligent Data Science & Artificial Intelligence research center, and Javi Creus, founder of Ideas for Change
Algorithms based on Artificial Intelligence (AI) have multiple applications and are currently capable of predicting who likes whom, what music you love, how much water a crop needs, when a traffic light should turn red, and how to adjust prices in a business, among countless other things we do every day that involve, many times transparently, the intervention of an artificial intelligence.
The Tik Tok proposal represents a significant paradigm shift that other social networks, such as Meta, will follow, moving us from a content recommendation system based on the individual's social networks to a system governed by an algorithm that proposes content based on a continuous test to maximize attention time.
Currently, artificial intelligence can recommend what temperature to set for waste combustion based on its composition, what combination of molecules can save you from a deadly disease, or how, in the advertisement from the DGT for Holy Week 2022, to anticipate who would die on the road during those holidays.
The Catalan Authority for Data Protection published a comprehensive report in 2020 reviewing cases of algorithms making automated decisions in various contexts in Catalonia [ACPD 2020] and highlighted the existence of countless cases in use in areas such as health, justice, education, mobility, banking, commerce, work, cybersecurity, communication, or society.
In legal matters, artificial intelligence algorithms could complement or even replace (although they should not) a regulation that can be consulted, is published in the Official Bulletin, and can be interpreted differently by various parties, or a decision-making body whose decisions are, in most cases, subject to appeal.
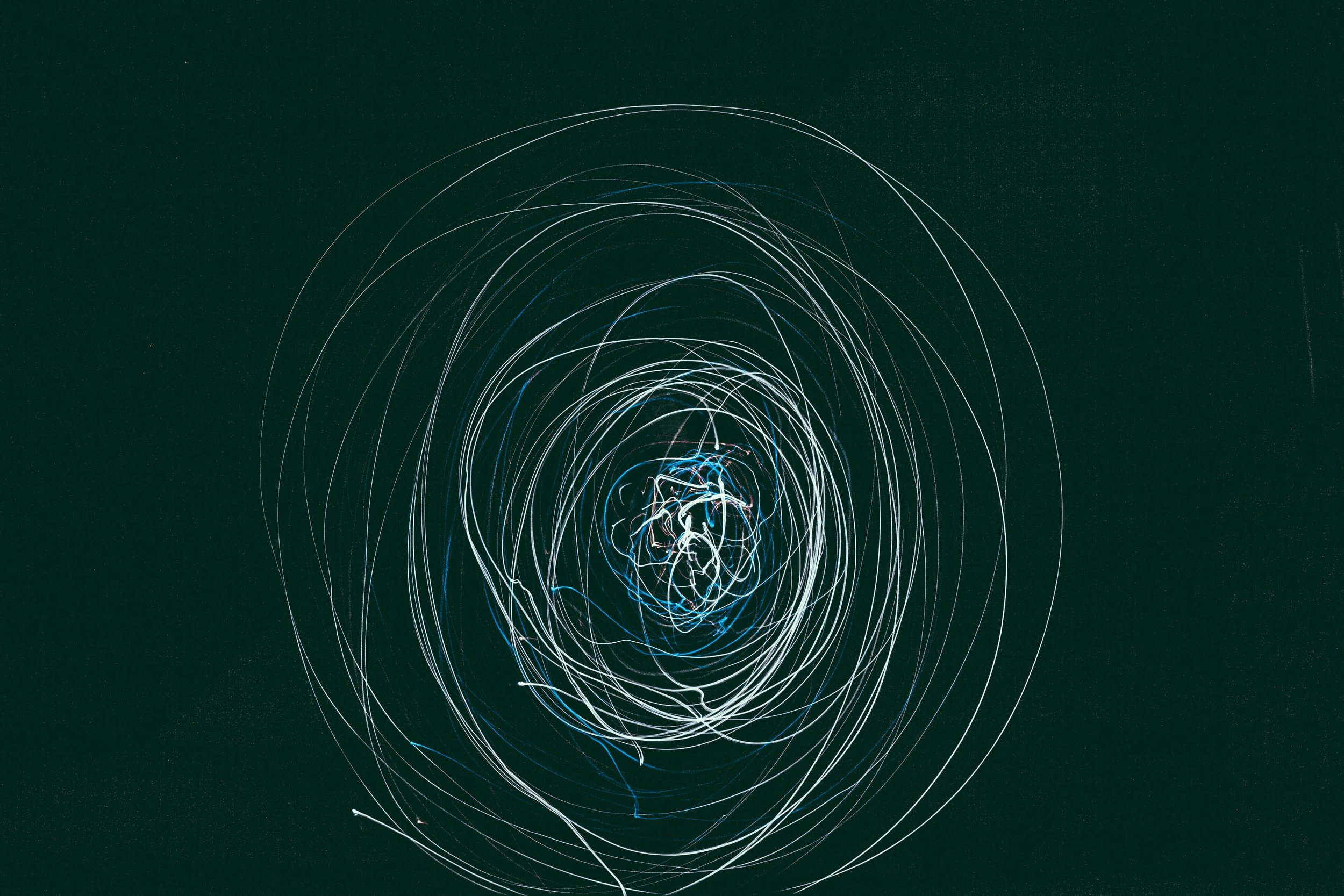
In the field of law [Bahena 2012], beyond intelligent document search engines relevant for resolving legal cases, which use AI for searches of legal precedents based on keywords, there are numerous cases of intelligent systems that support the drafting of claims, defenses, and even the ruling of judgments and their subsequent reasoning under different forms of AI ranging from classic rule-based reasoning systems (like GetAid, from the Australian government to determine access to legal advice on criminal and family matters) [Zeleznikov 2022], to the more advanced hybrid architectures that combine, for example, automatic reasoning with artificial neural networks (like Split-up, which proposes the division of assets and child custody in cases of separation and divorce in the Australian Court) [Zeleznikov 2004].
A close example is the request made by the Civio Foundation to access the source code of the BOSCO application, developed by the government and used by electricity companies to determine if a user in a vulnerable situation can receive the “social bonus,” that is, discounts on their energy bill. Despite verifying that many eligible applicants were not receiving this assistance, the request was denied in the first instance contrary to the Transparency Council's report, claiming it posed a danger to public safety (!). Civio has filed an appeal against this decision which leaves citizens unprotected against automated decisions that do not respect their rights.
It is important to note that the European Commission has undertaken decisive action to define a framework for the development of safe, ethical, and trustworthy AI, and is in the process of drafting the European AI Law (AI Act) [CE AI act 2021], which aims to ensure that this method in Europe will be oriented towards the common good, putting people at the center and respecting the fundamental rights of individuals, in contrast to the Chinese or North American vision, where data control is held respectively by the government (surveillance and social credit systems) or by the company owning the application that collects it (and monetizes it as it wishes).
Indeed, as early as 2018, pioneeringly, the EC drafted its ethical recommendations for safe and trustworthy AI (TrustWorthy AI, TWAI) [CE ethics 2018], dedicating the first of its axes to Human Agency and Human Oversight in a clear attempt to prevent AI-based applications from making autonomous decisions. That is, the place that Europe proposes to reserve for AI-based applications is that of an intelligent assistant for the user, who will effectively make the decision, such that there is always human validation of the recommendation, prediction, or diagnosis proposed by the algorithm.
On the other hand, in the Charter of Digital Rights (Catalan [CDDcat 2019] and Spanish [CDDSpain 2021]), the right of individuals to be informed of the intervention of an algorithm in any decision affecting them is recognized, and individuals also have the right to know the criteria with which the algorithm has evaluated them and how the result of that evaluation has been developed. This should allow for the detection of potential biases in the functioning of the algorithm that may eventually increase social inequalities or limit the rights of individuals.
Bias and Explainability
Among many others, known and rather scandalous cases of discrimination based on gender in AI-based algorithms evaluating funding applications across various channels are cited. Not least, Apple Card, the credit card launched by Apple in 2019, offered up to 20 times more liquidity and a longer repayment period to men than to women, even under equal conditions [Telford 2019]. The granting of credits to entrepreneurship has also been subject to scandalous comparative grievances for applications led by women in many countries, such as Chile [Montoya 2020], Turkey [Brock 2021], or even Italy [Alesina 2013], and even though the problem was detected as early as 2013, cases continued to arise in 2021.
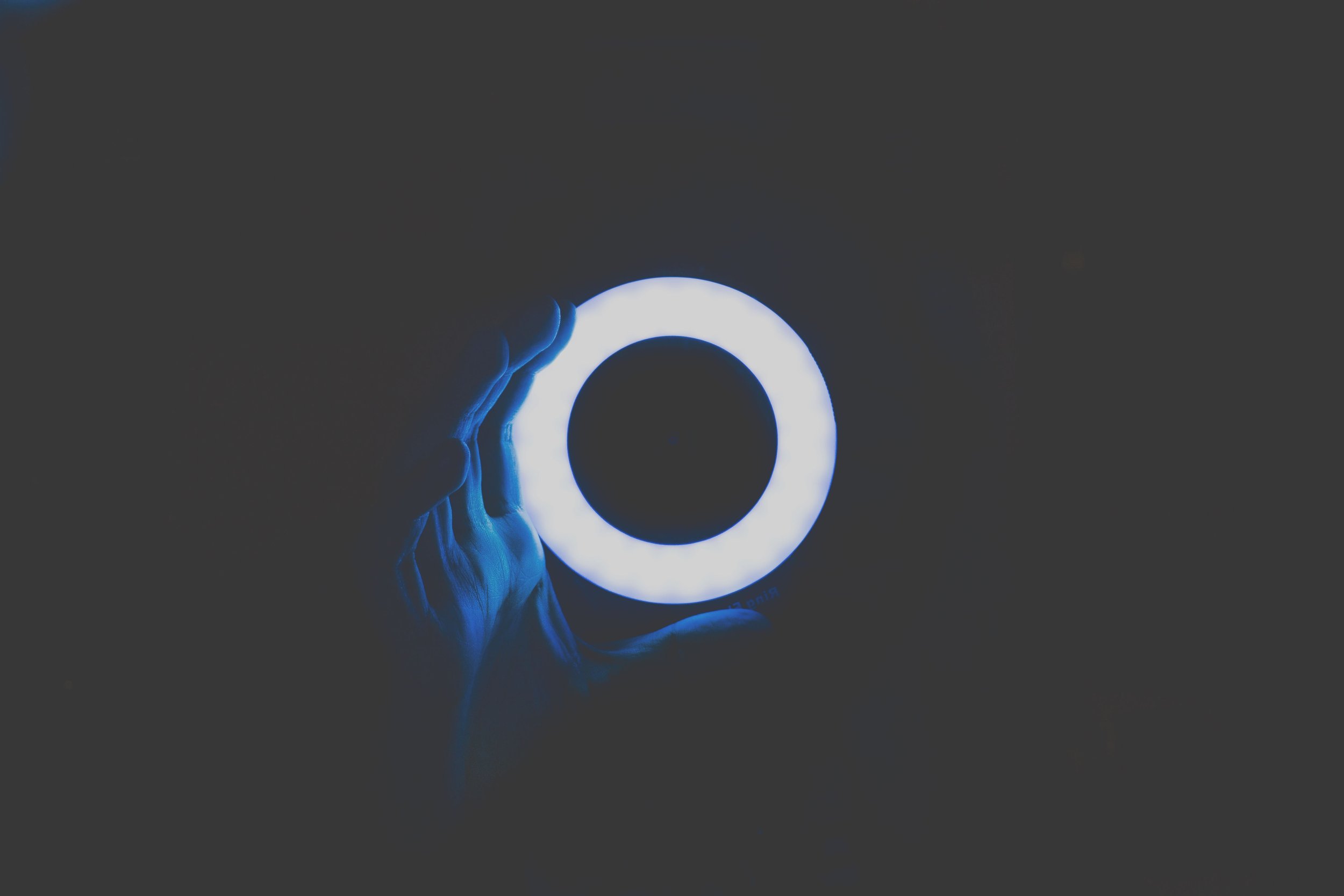
Preventing these types of situations directly impacts the type of algorithm that can be used to assist in decisions affecting individuals because they must be algorithms capable of explaining or arguing why they make a certain recommendation and not another or why they formulate a certain prediction. In fact, there is a relatively new branch of AI that has gained much traction, known as explainable AI, which addresses these issues. And so far, it is difficult to achieve that deep learning methods, which are making very good and very fast predictions about very complex realities, can adequately justify those predictions. This indeed occurs with all black-box algorithms, including not only those of deep learning but also all those based on artificial neural networks or evolutionary computation.
The application ChatGPT from Open AI, released to the general public on November 30, 2022, has a remarkable ability to draft all kinds of texts or lines of code on any topic it is questioned about; the barriers to the universalization of the electric car, drafting a standard rental contract, or the code for an application that makes an avatar wave hello only when there is a human in front of the screen are some of the topics it can provide answers about.
In all the tests conducted by the authors and many other users, the speed and plausibility of the responses offered by the application are surprising, although there are also observed absences of relevant content or arguments in specific fields that make it difficult to fully consider the results as reliable.
ChatGPT has been trained on millions of documents published up until 2021, but its creators have not revealed what documents they are. Millions of people experimenting with the application at this moment would like to know what the "universe" of knowledge used was to be able to interpret the possible biases in the results it offers.
Too Promising to Pass Up
It seems clear that the ability of algorithms to encompass complexity, bring us closer to a desired objectivity, but above all their capacity to generate economies of scale in activities related to knowledge represents an opportunity too attractive for progress to let go of, despite the known risks, and those others that we will discover in the coming years.
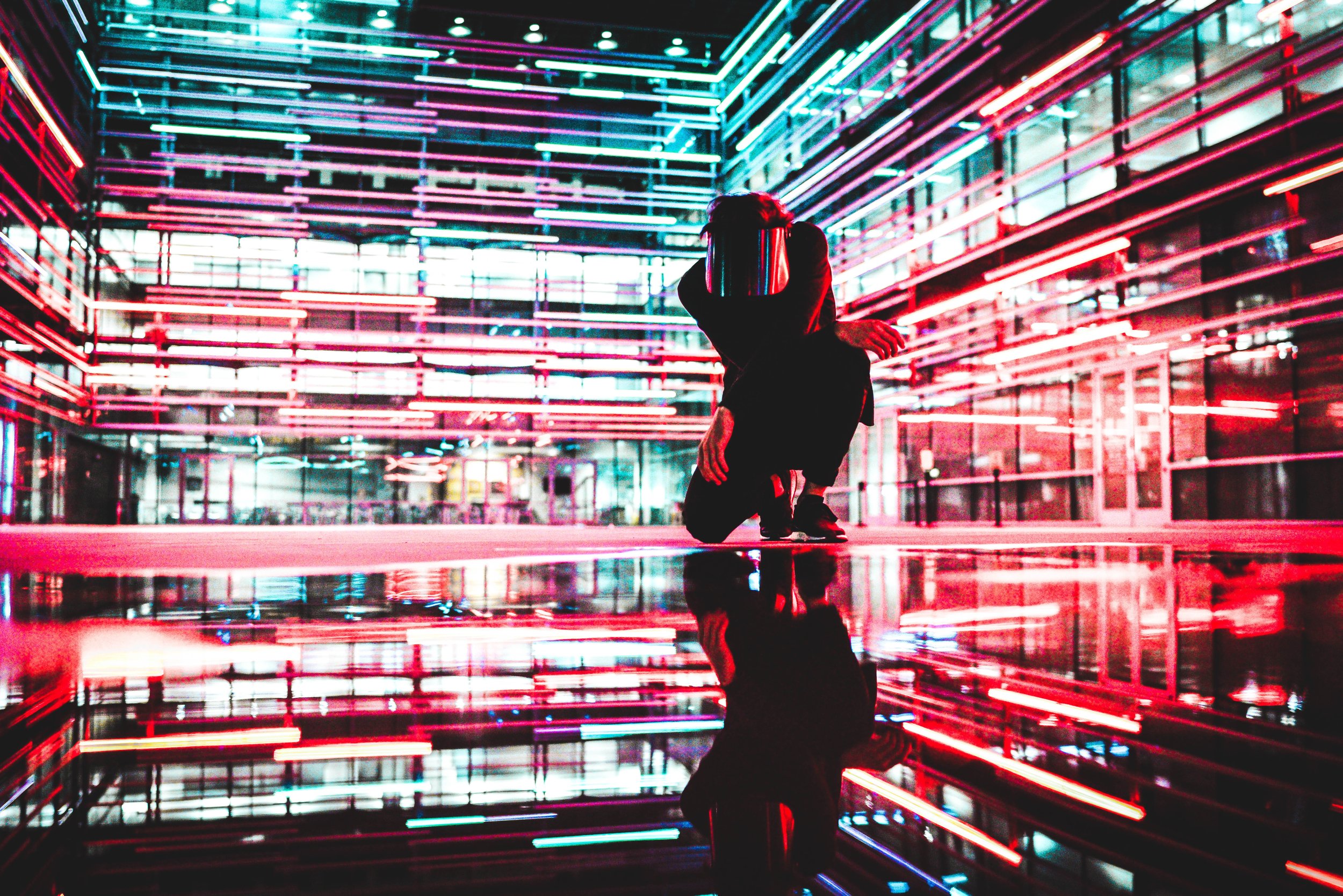
Only through algorithmic organization can the Hong Kong metro efficiently manage the ten thousand workers who each night perform the 2,600 repair and maintenance tasks necessary to provide public transport with extremely high punctuality levels (99.9% since 2014!) and generate savings of 2 days on repairs per week and about $800,000 per year [Chun 2005] [Hodson 2014]. The Barcelona metro has had an AI-based system since December 2020 that allows it to control the passenger flow of platforms and trains, and open or close access to them to create the safest conditions for passengers from the standpoint of virus spread [Nac 2020].
When an algorithm is encoded in a programming language understandable by machines, it becomes a tool that can scale its impact to a dimension unattainable through human-to-human communication. For example, the software update of a fleet of connected vehicles or robots allows incorporating improvements resulting from the group's learning to each of them. In parallel, each update of the algorithms managing our search or navigation tools opens and closes opportunities for businesses and citizens to discover each other.
In reality, we are witnessing the emerging development of a powerful technology, Artificial Intelligence, which, like all new things, generates certain fears and where good information can help dispel doubts. In this sense, the Catalan Artificial Intelligence Strategy [catalonia.ai 2021] launched a public course on Artificial Intelligence in 2021 aimed at providing basic training to the general public. The course, designed by the UPC research center, IDEAI (Intelligent Data Science and Artificial Intelligence research center), is free and can be accessed from the website https://ciutadanIA.cat.
Like all technologies, AI can present more or less ethical uses, with greater or lesser risk, and the current challenge is to find that delimitation in the uses of AI that allows us to take advantage of all of its benefits without exposing us to harm.
If we look back in history, fire or the knife are technologies that, when they appear, drastically change the history of humanity. Both, like AI and many others, have two sides. Fire allowed us to warm up and survive freezing temperatures, and also to cook, but if precautions are not taken, it can cause burns and fires that can lead to major natural disasters. The knife enabled new manipulations of food and the shaping of new tools contributing to the development of civilization, but it also serves to harm people; evidence of this is that in all cultures, we have developed rules that penalize the undesirable uses of these technologies. However, despite these risks, no one thinks to prohibit the manufacture and use of knives to protect us from their dangers and risks. And this is precisely what should also happen with Artificial Intelligence; it is more about identifying risks and regulating its uses to allow for beneficial development for all.
Trust, Machine Limits, and the Power of Data
If machine learning maximizes a function by applying computational power, speed, and the learning capacity of machines to a mass of data, then it is worth evaluating those dimensions in which machines are reliable and those situations in which the data mass is appropriate.
Then the question is: Who can we trust our "mass of data" to? Who can we trust to control the machines to work on our behalf?
The Edelman report 2022 indicates that globally we are at the lowest level of trust in businesses, NGOs, institutions, and the media since they began this series in the year 2000. The succession of financial crises, institutional corruption, fake news, and Covid-19 have installed distrust as the default sentiment towards institutions. The Dutch government as a whole was forced to resign on January 8, 2021, when it could be demonstrated that the SyRI system based on AI that had been used since 2014 to identify fraud among welfare beneficiaries suffered from a bias that only implicated immigrant families from vulnerable districts and had wrongly processed 26,000 families, forcing them to return subsidies unjustly. Hundreds of families suffering this unjust institutional harassment, causing depression, ruin, stigmatization, suicides, or imprisonment BY ERROR, because no one critically reviewed the algorithm's recommendations [Lazcoz 2022].
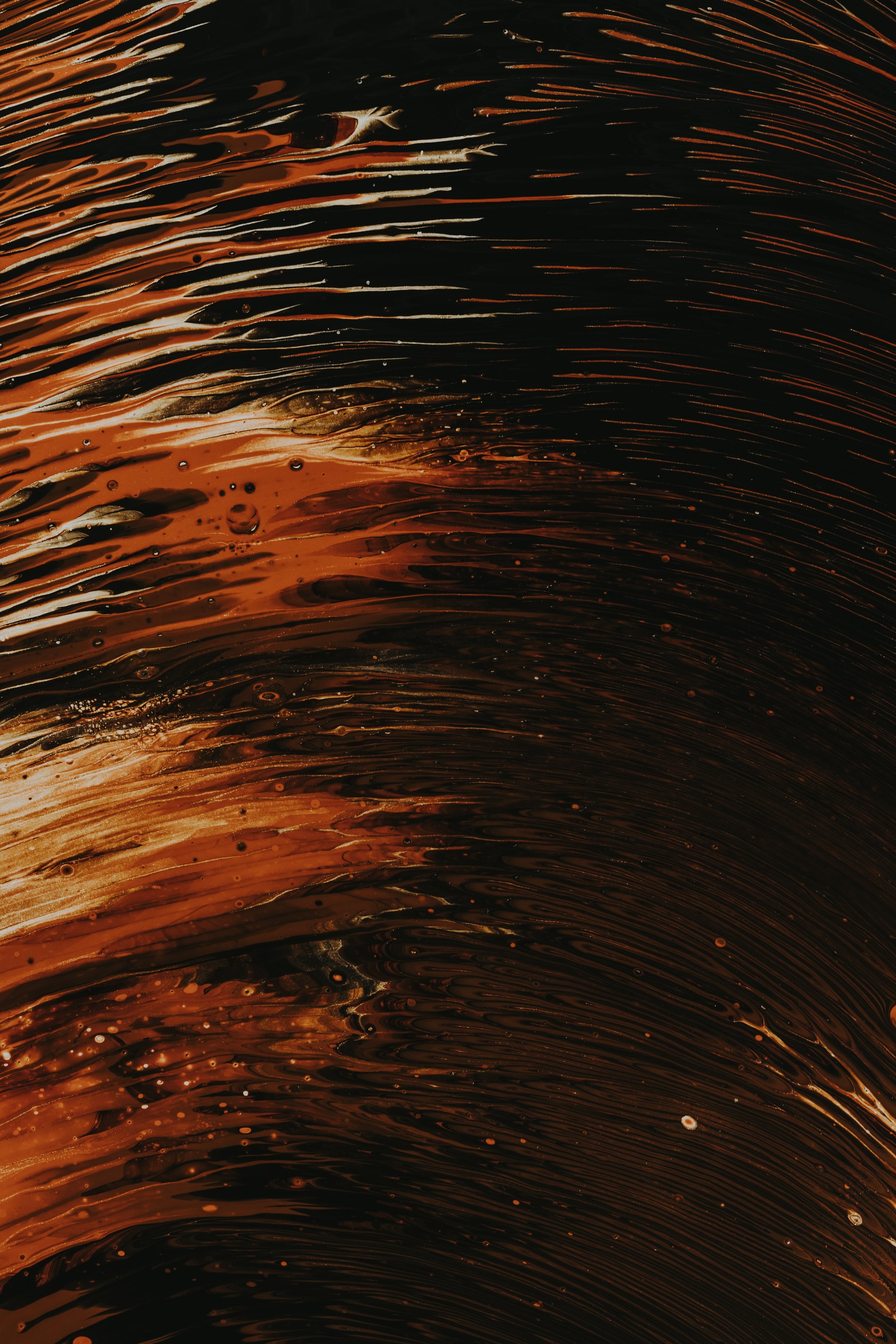
In the digital realm, Tim Berners Lee, the creator of the web, criticizes how his own creation, initially intended to be the greatest tool for knowledge democratization in history, has degenerated into an instrument of division and inequality through attention capture and behavior control and attempts to develop a better alternative.
On the other hand, the development of new technologies has led to a concentration of algorithms and data in the hands of a few global super-corporations that increasingly influence more aspects of our lives, with risks that this entails.
Ensuring that algorithms do not incorporate biases by design is one of the greatest challenges we face. In fact, the design of algorithms relies on the understanding the developer has of the real process being computationally represented, and to acquire this understanding, one must interact with the expert in that process and capture the relevant aspects to consider in the implementation.
In this transmission from the domain expert to the computing specialist, implicit knowledge plays many bad tricks. Neither the domain expert is aware that they have it, nor that they are using it in their reasoning, decisions, and actions, nor do they realize that they are not including it in their world description, nor are they transmitting it to the interlocutor, nor is the developer aware that they make (often dangerously oversimplifying) hypotheses that guide their implementation and can skew the algorithm's behavior.
A good part of implicit knowledge has a situated cultural component; that is, many social values are valid in a specific society or situation but are not universalizable, and some criteria are activated appropriately in humans vis-à-vis certain exceptional situations, but remain in the unconscious most of the time, and therefore, cannot be conveyed either to the verbal nor much less to the algorithm.
Machines can apply computational power, speed, and processing capability, but they are not sensitive to context, unless given a good formal description of it, they are incapable of dealing with exceptions if they have not been implemented to take them into account, nor are they capable of dealing with unforeseen events, unlike humans. This can lead to biased behaviors in algorithms that deal with very complex phenomena.
Such biases are not always intentional. Often we do not practice sufficiently the thorough analysis of the scenarios for which algorithms are built. Defining criteria for the majority, for the general case, is almost always a bad idea because it is in the exception, in the violation of the minority, where injustices appear.
It is necessary to employ more lateral thinking and conduct a more thorough analysis of the potential exceptional scenarios to reduce the bias in the algorithms' logic. Certainly, having diverse teams facilitates this task, as the combination of different perspectives on the same problem brings complementary views that naturally reduce logical biases, but also biases in the data.
On the other hand, algorithms are fed with data, and we have lost the good habit of using the old theory of sampling and experimental design to ensure that the data we use to train an AI will adequately represent the population under study and will not carry biases that will corrupt the predictions and recommendations of the resulting systems.
As explained by Kai Fu Lee in his book "AI Superpowers", the availability of data is more relevant than the algorithm's quality. For example, chess algorithms existed since 1983, but it wasn't until 1997 that Deep Blue defeated Kasparov, just six years after a database with 700,000 games between masters was published. In a world where basic algorithms are public, it is the availability of data that determines the advantage.
However, currently, data no longer only refers to numbers or measurable quantities that traditional statistics has been analyzing for so many years. Voice, images, videos, documents, tweets, opinions, our vital signs or values of virtual currencies are today invaluable sources of information from which to extract relevant information in all directions and constitute a new generation of complex data that are extensively exploited from the realm of artificial intelligence. Video games, virtual simulations, or the much-anticipated metaverse are all built with data and represent computational metaphors of real or imagined worlds, and as Beau Cronin states, they may be preferable to the real world for those who do not enjoy the "privilege of reality": it is striking to note that nowadays 50% of young people already believe they have more life opportunities in the online environment than in the real world.
Decentralized or distributed data architectures, along with the emerging technologies of federated data science, are part of an intense debate about how to develop data policies, which not only address the support architectures for storing data but also how they are produced, policies for data openness and ownership models (commercial or institutional?) and use licenses. Some argue that if the production of data is distributed - the facial recognition algorithm "facelift" from Google is based on photographs of 82,000 people - its ownership should also be distributed. In some cases, the courts have also mandated the destruction of algorithms generated through the misleading acquisition of data, like the Kurbo application that captured data from eight-year-old children without their guardians' knowledge.
Challenges and Proposals
There is no longer any doubt that human activity modifies living conditions on the planet and that it has done so at an accelerated pace over the last two centuries, to the point of making us aware of the climate and social emergency we live in.
Our collective challenge now is life: how to generate conditions for a decent life for the 8 billion people inhabiting the planet, and how to do so without our survival threatening the quantity, quality, and diversity of life of other living beings or future generations.
We cannot do without Artificial Intelligence as a tool to encompass the complexity, simultaneity, and scale of these challenges, but as we have argued earlier, we also cannot let that which is technically possible, although not necessarily desirable, guide us in its development.
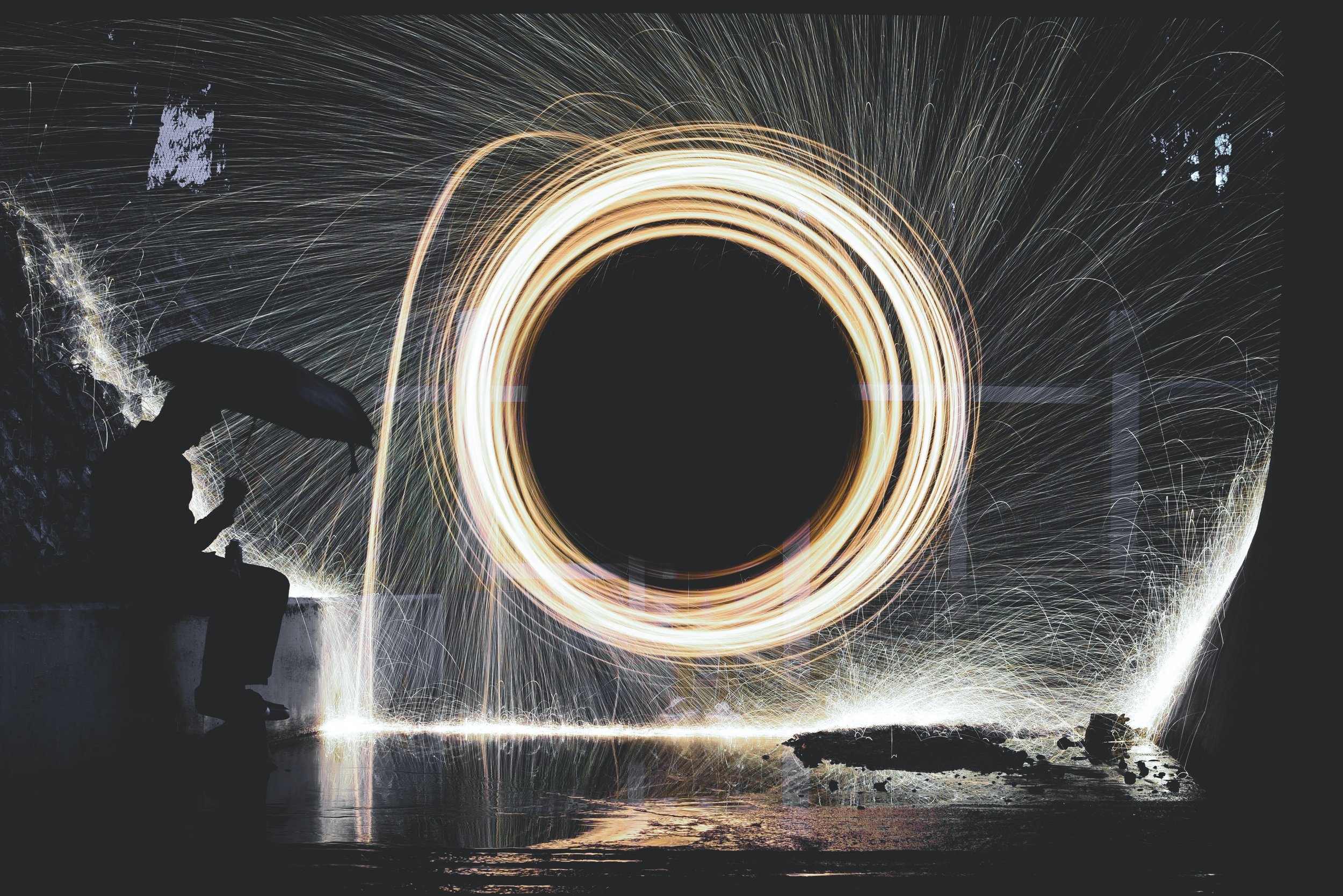
Only through algorithmic organization can the Hong Kong metro efficiently manage the ten thousand workers who each night perform the 2,600 repair and maintenance tasks necessary to provide public transport with extremely high punctuality levels (99.9% since 2014!) and generate savings of 2 days on repairs per week and about $800,000 per year [Chun 2005] [Hodson 2014]. The Barcelona metro has had an AI-based system since December 2020 that allows it to control the passenger flow of platforms and trains, and open or close access to them to create the safest conditions for passengers from the standpoint of virus spread [Nac 2020].
When an algorithm is encoded in a programming language understandable by machines, it becomes a tool that can scale its impact to a dimension unattainable through human-to-human communication. For example, the software update of a fleet of connected vehicles or robots allows incorporating improvements resulting from the group's learning to each of them. In parallel, each update of the algorithms managing our search or navigation tools opens and closes opportunities for businesses and citizens to discover each other.
In reality, we are witnessing the emerging development of a powerful technology, Artificial Intelligence, which, like all new things, generates certain fears and where good information can help dispel doubts. In this sense, the Catalan Artificial Intelligence Strategy [catalonia.ai 2021] launched a public course on Artificial Intelligence in 2021 aimed at providing basic training to the general public. The course, designed by the UPC research center, IDEAI (Intelligent Data Science and Artificial Intelligence research center), is free and can be accessed from the website https://ciutadanIA.cat.
Like all technologies, AI can present more or less ethical uses, with greater or lesser risk, and the current challenge is to find that delimitation in the uses of AI that allows us to take advantage of all its benefits without exposing us to harm.
If we look back in history, fire or the knife are technologies that, when they appear, drastically change the history of humanity. Both, like AI and many others, have two sides. Fire allowed us to warm up and survive freezing temperatures, and also to cook, but if precautions are not taken, it can cause burns and fires that can lead to major natural disasters. The knife enabled new manipulations of food and the shaping of new tools contributing to the development of civilization, but it also serves to harm people; evidence of this is that in all cultures, we have developed rules that penalize the undesirable uses of these technologies. However, despite these risks, no one thinks to prohibit the manufacture and use of knives to protect us from their dangers and risks. And this is precisely what should also happen with Artificial Intelligence; it is more about identifying risks and regulating its uses to allow for beneficial development for all.
Trust, Machine Limits, and the Power of Data
If machine learning maximizes a function by applying computational power, speed, and the learning capacity of machines to a mass of data, then it is worth evaluating those dimensions in which machines are reliable and those situations in which the data mass is appropriate.
Then the question is: Who can we trust our "mass of data" to? Who can we trust to control the machines to work on our behalf?
The Edelman report 2022 indicates that globally we are at the lowest level of trust in businesses, NGOs, institutions, and the media since they began this series in the year 2000. The succession of financial crises, institutional corruption, fake news, and Covid-19 have installed distrust as the default sentiment toward institutions. The Dutch government as a whole was forced to resign on January 8, 2021, when it could be demonstrated that the SyRI system based on AI that had been used since 2014 to identify fraud among welfare beneficiaries suffered from a bias that only implicated immigrant families from vulnerable districts and had wrongly processed 26,000 families, forcing them to return subsidies unjustly. Hundreds of families suffering this unjust institutional harassment, causing depression, ruin, stigmatization, suicides, or imprisonment BY ERROR, because no one critically reviewed the algorithm's recommendations [Lazcoz 2022].
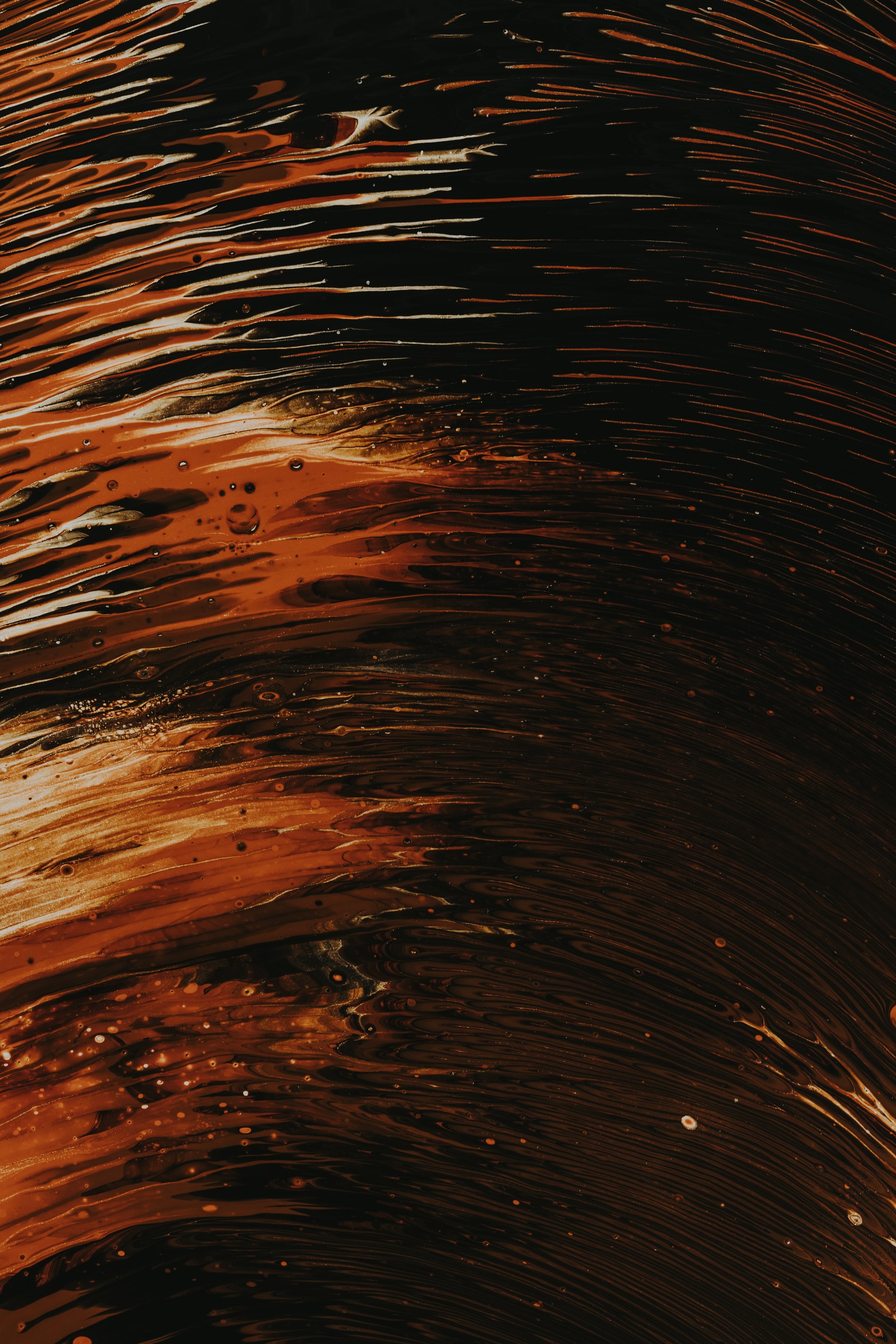
In the digital realm, Tim Berners Lee, the creator of the web, criticizes how his own creation, initially intended to be the greatest tool for knowledge democratization in history, has degenerated into an instrument of division and inequality through attention capture and behavior control and attempts to develop a better alternative.
On the other hand, the development of new technologies has led to a concentration of algorithms and data in the hands of a few global super-corporations that increasingly influence more aspects of our lives, with risks that this entails.
Ensuring that algorithms do not incorporate biases by design is one of the greatest challenges we face. In fact, the design of algorithms relies on the understanding the developer has of the real process being computationally represented, and to acquire this understanding, one must interact with the expert in that process and capture the relevant aspects to consider in the implementation.
In this transmission from the domain expert to the computing specialist, implicit knowledge plays many bad tricks. Neither the domain expert is aware that they have it, nor that they are using it in their reasoning, decisions, and actions, nor do they realize that they are not including it in their world description, nor are they transmitting it to the interlocutor, nor is the developer aware that they make (often dangerously oversimplifying) hypotheses that guide their implementation and can skew the algorithm's behavior.
A good part of implicit knowledge has a situated cultural component; that is, many social values are valid in a specific society or situation but are not universalizable, and some criteria are activated appropriately in humans vis-à-vis certain exceptional situations, but remain in the unconscious most of the time, and therefore, cannot be conveyed either to the verbal nor much less to the algorithm.
Machines can apply computational power, speed, and processing capability, but they are not sensitive to context, unless given a good formal description of it, they are incapable of dealing with exceptions if they have not been implemented to take them into account, nor are they capable of dealing with unforeseen events, unlike humans. This can lead to biased behaviors in algorithms that deal with very complex phenomena.
Such biases are not always intentional. Often we do not practice sufficiently the thorough analysis of the scenarios for which algorithms are built. Defining criteria for the majority, for the general case, is almost always a bad idea because it is in the exception, in the violation of the minority, where injustices appear.
It is necessary to employ more lateral thinking and conduct a more thorough analysis of the potential exceptional scenarios to reduce the bias in the algorithms' logic. Certainly, having diverse teams facilitates this task, as the combination of different perspectives on the same problem brings complementary views that naturally reduce logical biases, but also biases in the data.
On the other hand, algorithms are fed with data, and we have lost the good habit of using the old theory of sampling and experimental design to ensure that the data we use to train an AI will adequately represent the population under study and will not carry biases that will corrupt the predictions and recommendations of the resulting systems.
As explained by Kai Fu Lee in his book "AI Superpowers", the availability of data is more relevant than the algorithm's quality. For example, chess algorithms existed since 1983, but it wasn't until 1997 that Deep Blue defeated Kasparov, just six years after a database with 700,000 games between masters was published. In a world where basic algorithms are public, it is the availability of data that determines the advantage.
However, currently, data no longer only refers to numbers or measurable quantities that traditional statistics has been analyzing for so many years. Voice, images, videos, documents, tweets, opinions, our vital signs or values of virtual currencies are today invaluable sources of information from which to extract relevant information in all directions and constitute a new generation of complex data that are extensively exploited from the realm of artificial intelligence. Video games, virtual simulations, or the much-anticipated metaverse are all built with data and represent computational metaphors of real or imagined worlds, and as Beau Cronin states, they may be preferable to the real world for those who do not enjoy the "privilege of reality": it is striking to note that nowadays 50% of young people already believe they have more life opportunities in the online environment than in the real world.
Decentralized or distributed data architectures, along with the emerging technologies of federated data science, are part of an intense debate about how to develop data policies, which not only address the support architectures for storing data but also how they are produced, policies for data openness and ownership models (commercial or institutional?) and use licenses. Some argue that if the production of data is distributed - the facial recognition algorithm "facelift" from Google is based on photographs of 82,000 people - its ownership should also be distributed. In some cases, the courts have also mandated the destruction of algorithms generated through the misleading acquisition of data, like the Kurbo application that captured data from eight-year-old children without their guardians' knowledge.
Challenges and Proposals
There is no longer any doubt that human activity modifies living conditions on the planet and that it has done so at an accelerated pace over the last two centuries, to the point of making us aware of the climate and social emergency we live in.
Our collective challenge now is life: how to generate conditions for a decent life for the 8 billion people inhabiting the planet, and how to do so without our survival threatening the quantity, quality, and diversity of life of other living beings or future generations.
We cannot do without Artificial Intelligence as a tool to encompass the complexity, simultaneity, and scale of these challenges, but as we have argued earlier, we also cannot let that which is technically possible, although not necessarily desirable, guide us in its development.
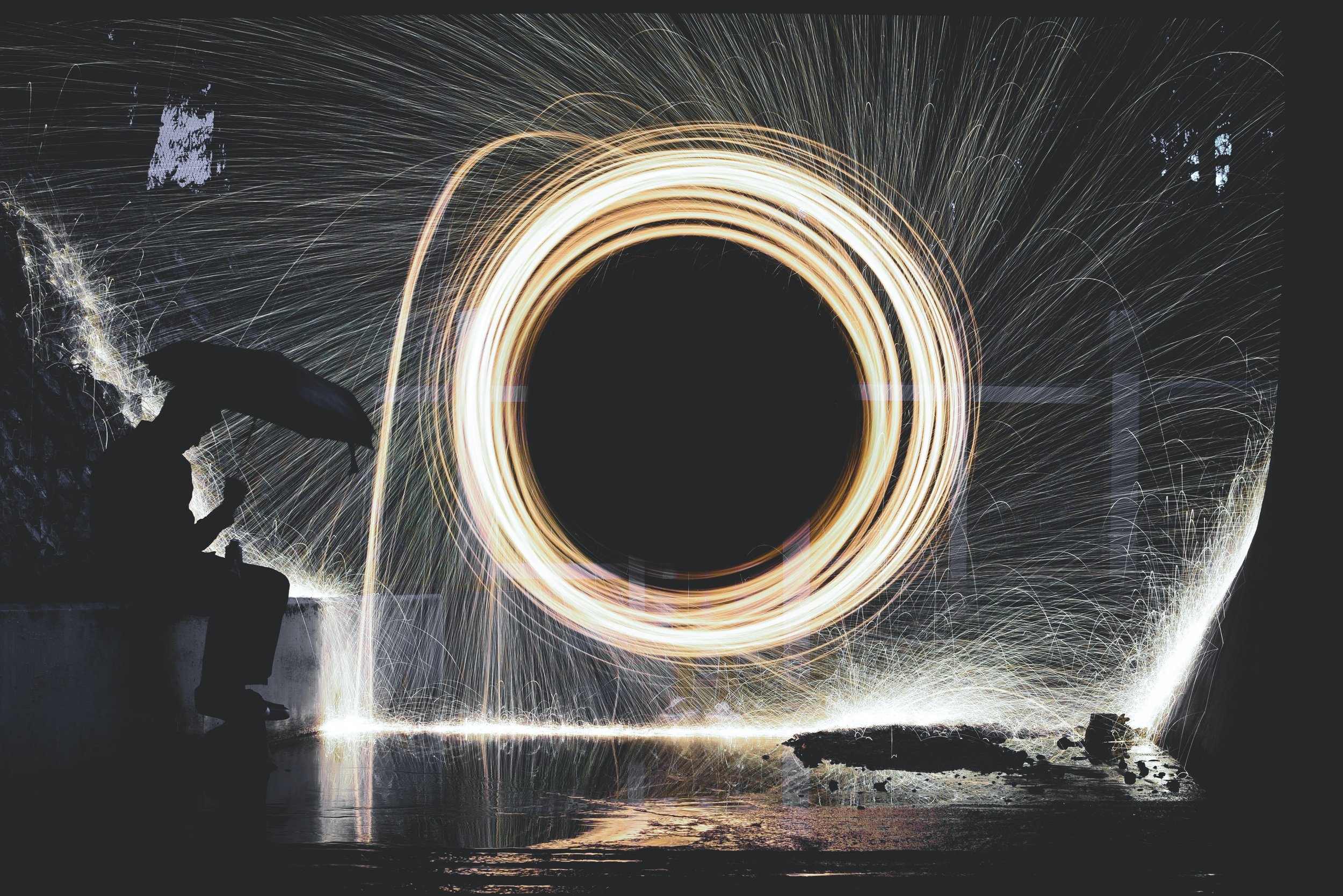
Only through algorithmic organization can the Hong Kong metro efficiently manage the ten thousand workers who each night perform the 2,600 repair and maintenance tasks necessary to provide public transport with extremely high punctuality levels (99.9% since 2014!) and generate savings of 2 days on repairs per week and about $800,000 per year [Chun 2005] [Hodson 2014]. The Barcelona metro has had an AI-based system since December 2020 that allows it to control the passenger flow of platforms and trains, and open or close access to them to create the safest conditions for passengers from the standpoint of virus spread [Nac 2020].
When an algorithm is encoded in a programming language understandable by machines, it becomes a tool that can scale its impact to a dimension unattainable through human-to-human communication. For example, the software update of a fleet of connected vehicles or robots allows incorporating improvements resulting from the group's learning to each of them. In parallel, each update of the algorithms managing our search or navigation tools opens and closes opportunities for businesses and citizens to discover each other.
In reality, we are witnessing the emerging development of a powerful technology, Artificial Intelligence, which, like all new things, generates certain fears and where good information can help dispel doubts. In this sense, the Catalan Artificial Intelligence Strategy [catalonia.ai 2021] launched a public course on Artificial Intelligence in 2021 aimed at providing basic training to the general public. The course, designed by the UPC research center, IDEAI (Intelligent Data Science and Artificial Intelligence research center), is free and can be accessed from the website https://ciutadanIA.cat.
Like all technologies, AI can present more or less ethical uses, with greater or lesser risk, and the current challenge is to find that delimitation in the uses of AI that allows us to take advantage of all its benefits without exposing us to harm.
If we look back in history, fire or the knife are technologies that, when they appear, drastically change the history of humanity. Both, like AI and many others, have two sides. Fire allowed us to warm up and survive freezing temperatures, and also to cook, but if precautions are not taken, it can cause burns and fires that can lead to major natural disasters. The knife enabled new manipulations of food and the shaping of new tools contributing to the development of civilization, but it also serves to harm people; evidence of this is that in all cultures, we have developed rules that penalize the undesirable uses of these technologies. However, despite these risks, no one thinks to prohibit the manufacture and use of knives to protect us from their dangers and risks. And this is precisely what should also happen with Artificial Intelligence; it is more about identifying risks and regulating its uses to allow for beneficial development for all.
Trust, Machine Limits, and the Power of Data
If machine learning maximizes a function by applying computational power, speed, and the learning capacity of machines to a mass of data, then it is worth evaluating those dimensions in which machines are reliable and those situations in which the data mass is appropriate.
Then the question is: Who can we trust our "mass of data" to? Who can we trust to control the machines to work on our behalf?
The Edelman report 2022 indicates that globally we are at the lowest level of trust in businesses, NGOs, institutions, and the media since they began this series in the year 2000. The succession of financial crises, institutional corruption, fake news, and Covid-19 have installed distrust as the default sentiment toward institutions. The Dutch government as a whole was forced to resign on January 8, 2021, when it could be demonstrated that the SyRI system based on AI that had been used since 2014 to identify fraud among welfare beneficiaries suffered from a bias that only implicated immigrant families from vulnerable districts and had wrongly processed 26,000 families, forcing them to return subsidies unjustly. Hundreds of families suffering this unjust institutional harassment, causing depression, ruin, stigmatization, suicides, or imprisonment BY ERROR, because no one critically reviewed the algorithm's recommendations [Lazcoz 2022].
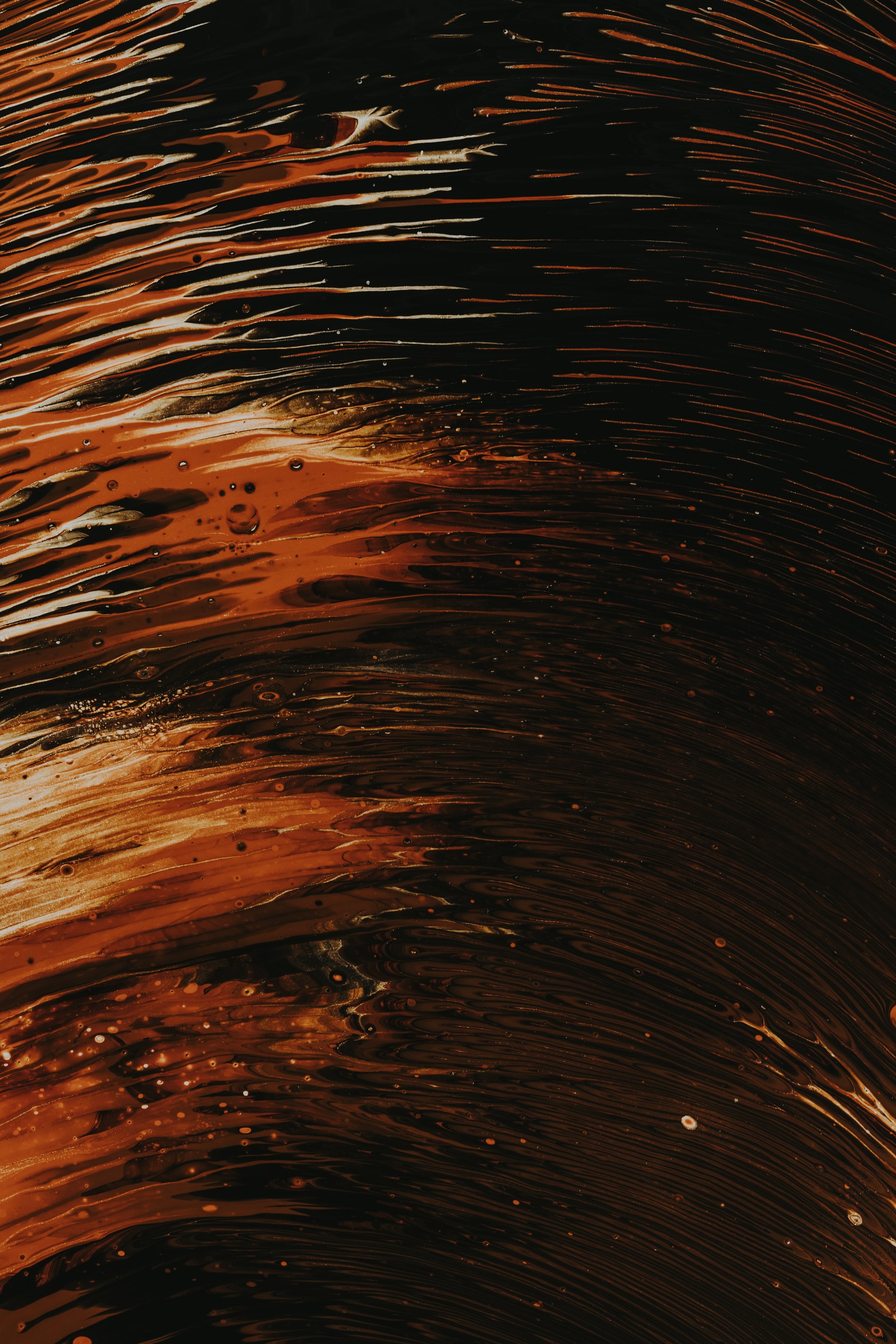
In the digital realm, Tim Berners Lee, the creator of the web, criticizes how his own creation, initially intended to be the greatest tool for knowledge democratization in history, has degenerated into an instrument of division and inequality through attention capture and behavior control and attempts to develop a better alternative.
On the other hand, the development of new technologies has led to a concentration of algorithms and data in the hands of a few global super-corporations that increasingly influence more aspects of our lives, with risks that this entails.
Ensuring that algorithms do not incorporate biases by design is one of the greatest challenges we face. In fact, the design of algorithms relies on the understanding the developer has of the real process being computationally represented, and to acquire this understanding, one must interact with the expert in that process and capture the relevant aspects to consider in the implementation.
In this transmission from the domain expert to the computing specialist, implicit knowledge plays many bad tricks. Neither the domain expert is aware that they have it, nor that they are using it in their reasoning, decisions, and actions, nor do they realize that they are not including it in their world description, nor are they transmitting it to the interlocutor, nor is the developer aware that they make (often dangerously oversimplifying) hypotheses that guide their implementation and can skew the algorithm's behavior.
A good part of implicit knowledge has a situated cultural component; that is, many social values are valid in a specific society or situation but are not universalizable, and some criteria are activated appropriately in humans vis-à-vis certain exceptional situations, but remain in the unconscious most of the time, and therefore, cannot be conveyed either to the verbal nor much less to the algorithm.
Machines can apply computational power, speed, and processing capability, but they are not sensitive to context, unless given a good formal description of it, they are incapable of dealing with exceptions if they have not been implemented to take them into account, nor are they capable of dealing with unforeseen events, unlike humans. This can lead to biased behaviors in algorithms that deal with very complex phenomena.
Such biases are not always intentional. Often we do not practice sufficiently the thorough analysis of the scenarios for which algorithms are built. Defining criteria for the majority, for the general case, is almost always a bad idea because it is in the exception, in the violation of the minority, where injustices appear.
It is necessary to employ more lateral thinking and conduct a more thorough analysis of the potential exceptional scenarios to reduce the bias in the algorithms' logic. Certainly, having diverse teams facilitates this task, as the combination of different perspectives on the same problem brings complementary views that naturally reduce logical biases, but also biases in the data.
On the other hand, algorithms are fed with data, and we have lost the good habit of using the old theory of sampling and experimental design to ensure that the data we use to train an AI will adequately represent the population under study and will not carry biases that will corrupt the predictions and recommendations of the resulting systems.
As explained by Kai Fu Lee in his book "AI Superpowers", the availability of data is more relevant than the algorithm's quality. For example, chess algorithms existed since 1983, but it wasn't until 1997 that Deep Blue defeated Kasparov, just six years after a database with 700,000 games between masters was published. In a world where basic algorithms are public, it is the availability of data that determines the advantage.
However, currently, data no longer only refers to numbers or measurable quantities that traditional statistics has been analyzing for so many years. Voice, images, videos, documents, tweets, opinions, our vital signs or values of virtual currencies are today invaluable sources of information from which to extract relevant information in all directions and constitute a new generation of complex data that are extensively exploited from the realm of artificial intelligence. Video games, virtual simulations, or the much-anticipated metaverse are all built with data and represent computational metaphors of real or imagined worlds, and as Beau Cronin states, they may be preferable to the real world for those who do not enjoy the "privilege of reality": it is striking to note that nowadays 50% of young people already believe they have more life opportunities in the online environment than in the real world.
Decentralized or distributed data architectures, along with the emerging technologies of federated data science, are part of an intense debate about how to develop data policies, which not only address the support architectures for storing data but also how they are produced, policies for data openness and ownership models (commercial or institutional?) and use licenses. Some argue that if the production of data is distributed - the facial recognition algorithm "facelift" from Google is based on photographs of 82,000 people - its ownership should also be distributed. In some cases, the courts have also mandated the destruction of algorithms generated through the misleading acquisition of data, like the Kurbo application that captured data from eight-year-old children without their guardians' knowledge.
Challenges and Proposals
There is no longer any doubt that human activity modifies living conditions on the planet and that it has done so at an accelerated pace over the last two centuries, to the point of making us aware of the climate and social emergency we live in.
Our collective challenge now is life: how to generate conditions for a decent life for the 8 billion people inhabiting the planet, and how to do so without our survival threatening the quantity, quality, and diversity of life of other living beings or future generations.
We cannot do without Artificial Intelligence as a tool to encompass the complexity, simultaneity, and scale of these challenges, but as we have argued earlier, we also cannot let that which is technically possible, although not necessarily desirable, guide us in its development.
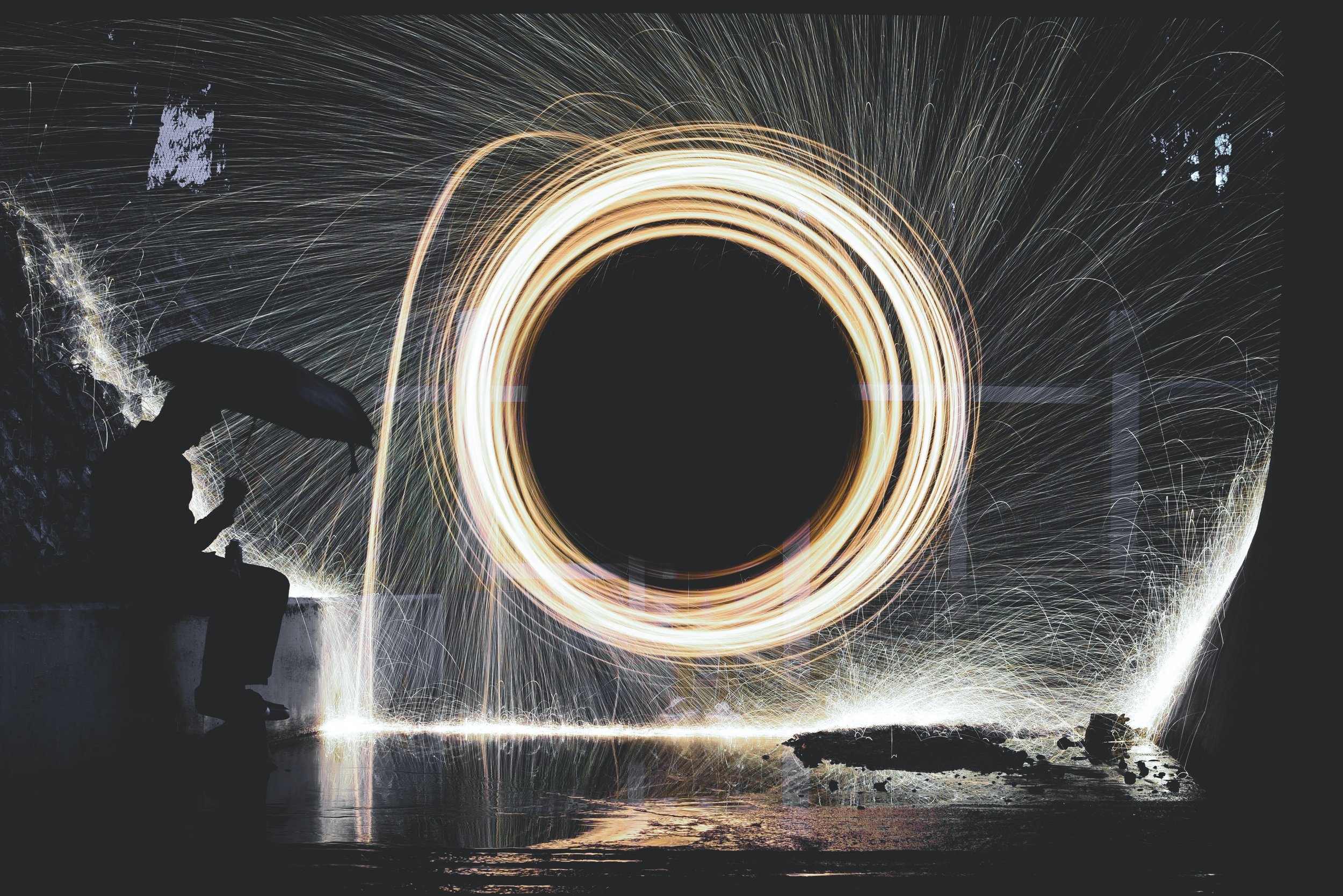
By Karina Gibert, director of the Intelligent Data Science & Artificial Intelligence research center, and Javi Creus, founder of Ideas for Change
Algorithms based on Artificial Intelligence (AI) have multiple applications and are currently capable of predicting who likes whom, what music you love, how much water a crop needs, when a traffic light should turn red, and how to adjust prices in a business, among countless other things we do every day that involve, many times transparently, the intervention of an artificial intelligence.
The Tik Tok proposal represents a significant paradigm shift that other social networks, such as Meta, will follow, moving us from a content recommendation system based on the individual's social networks to a system governed by an algorithm that proposes content based on a continuous test to maximize attention time.
Currently, artificial intelligence can recommend what temperature to set for waste combustion based on its composition, what combination of molecules can save you from a deadly disease, or how, in the advertisement from the DGT for Holy Week 2022, to anticipate who would die on the road during those holidays.
The Catalan Authority for Data Protection published a comprehensive report in 2020 reviewing cases of algorithms making automated decisions in various contexts in Catalonia [ACPD 2020] and highlighted the existence of countless cases in use in areas such as health, justice, education, mobility, banking, commerce, work, cybersecurity, communication, or society.
In legal matters, artificial intelligence algorithms could complement or even replace (although they should not) a regulation that can be consulted, is published in the Official Bulletin, and can be interpreted differently by various parties, or a decision-making body whose decisions are, in most cases, subject to appeal.
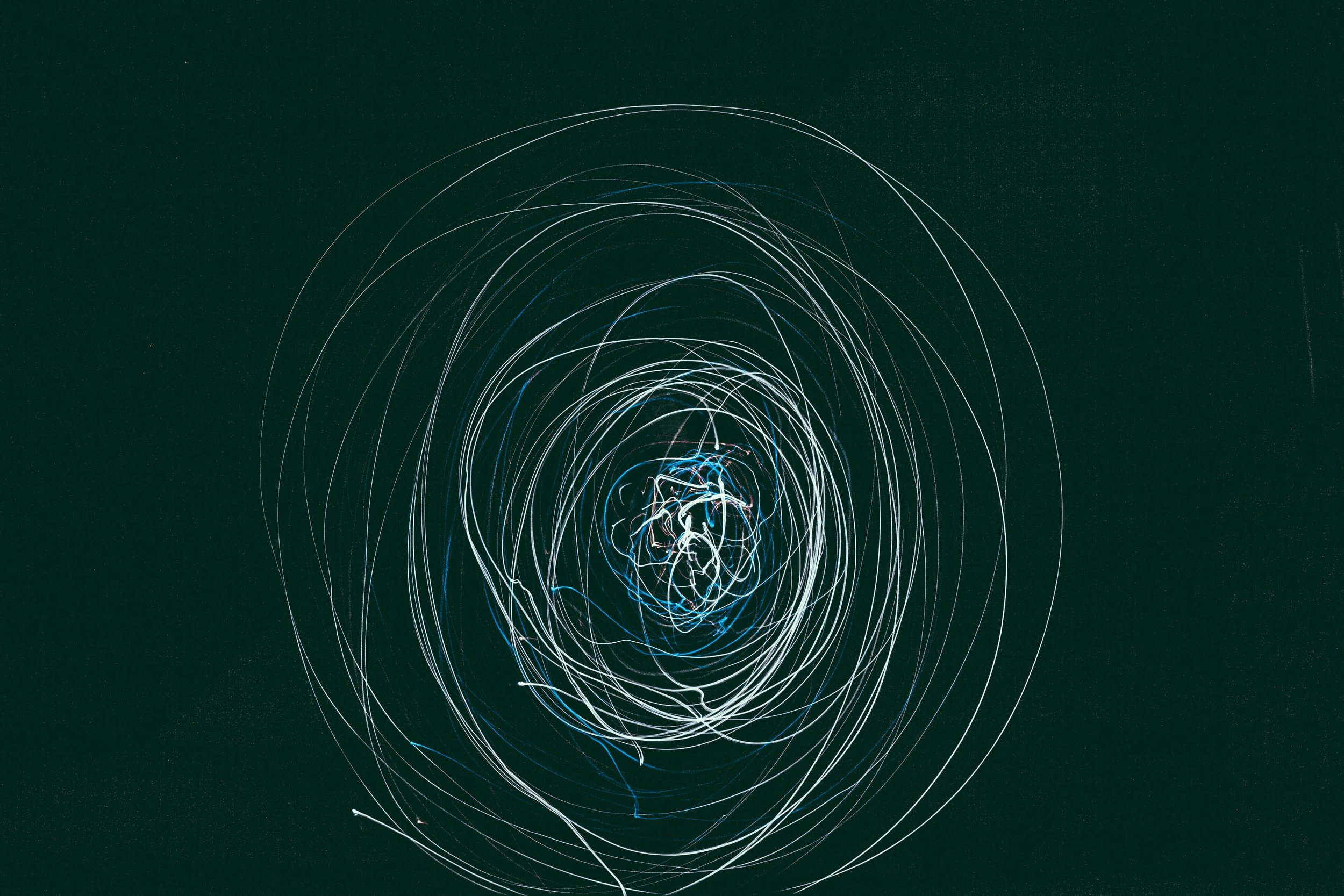
In the field of law [Bahena 2012], beyond intelligent document search engines relevant for resolving legal cases, which use AI for searches of legal precedents based on keywords, there are numerous cases of intelligent systems that support the drafting of claims, defenses, and even the ruling of judgments and their subsequent reasoning under different forms of AI ranging from classic rule-based reasoning systems (like GetAid, from the Australian government to determine access to legal advice on criminal and family matters) [Zeleznikov 2022], to the more advanced hybrid architectures that combine, for example, automatic reasoning with artificial neural networks (like Split-up, which proposes the division of assets and child custody in cases of separation and divorce in the Australian Court) [Zeleznikov 2004].
A close example is the request made by the Civio Foundation to access the source code of the BOSCO application, developed by the government and used by electricity companies to determine if a user in a vulnerable situation can receive the “social bonus,” that is, discounts on their energy bill. Despite verifying that many eligible applicants were not receiving this assistance, the request was denied in the first instance contrary to the Transparency Council's report, claiming it posed a danger to public safety (!). Civio has filed an appeal against this decision which leaves citizens unprotected against automated decisions that do not respect their rights.
It is important to note that the European Commission has undertaken decisive action to define a framework for the development of safe, ethical, and trustworthy AI, and is in the process of drafting the European AI Law (AI Act) [CE AI act 2021], which aims to ensure that this method in Europe will be oriented towards the common good, putting people at the center and respecting the fundamental rights of individuals, in contrast to the Chinese or North American vision, where data control is held respectively by the government (surveillance and social credit systems) or by the company owning the application that collects it (and monetizes it as it wishes).
Indeed, as early as 2018, pioneeringly, the EC drafted its ethical recommendations for safe and trustworthy AI (TrustWorthy AI, TWAI) [CE ethics 2018], dedicating the first of its axes to Human Agency and Human Oversight in a clear attempt to prevent AI-based applications from making autonomous decisions. That is, the place that Europe proposes to reserve for AI-based applications is that of an intelligent assistant for the user, who will effectively make the decision, such that there is always human validation of the recommendation, prediction, or diagnosis proposed by the algorithm.
On the other hand, in the Charter of Digital Rights (Catalan [CDDcat 2019] and Spanish [CDDSpain 2021]), the right of individuals to be informed of the intervention of an algorithm in any decision affecting them is recognized, and individuals also have the right to know the criteria with which the algorithm has evaluated them and how the result of that evaluation has been developed. This should allow for the detection of potential biases in the functioning of the algorithm that may eventually increase social inequalities or limit the rights of individuals.
Bias and Explainability
Among many others, known and rather scandalous cases of discrimination based on gender in AI-based algorithms evaluating funding applications across various channels are cited. Not least, Apple Card, the credit card launched by Apple in 2019, offered up to 20 times more liquidity and a longer repayment period to men than to women, even under equal conditions [Telford 2019]. The granting of credits to entrepreneurship has also been subject to scandalous comparative grievances for applications led by women in many countries, such as Chile [Montoya 2020], Turkey [Brock 2021], or even Italy [Alesina 2013], and even though the problem was detected as early as 2013, cases continued to arise in 2021.
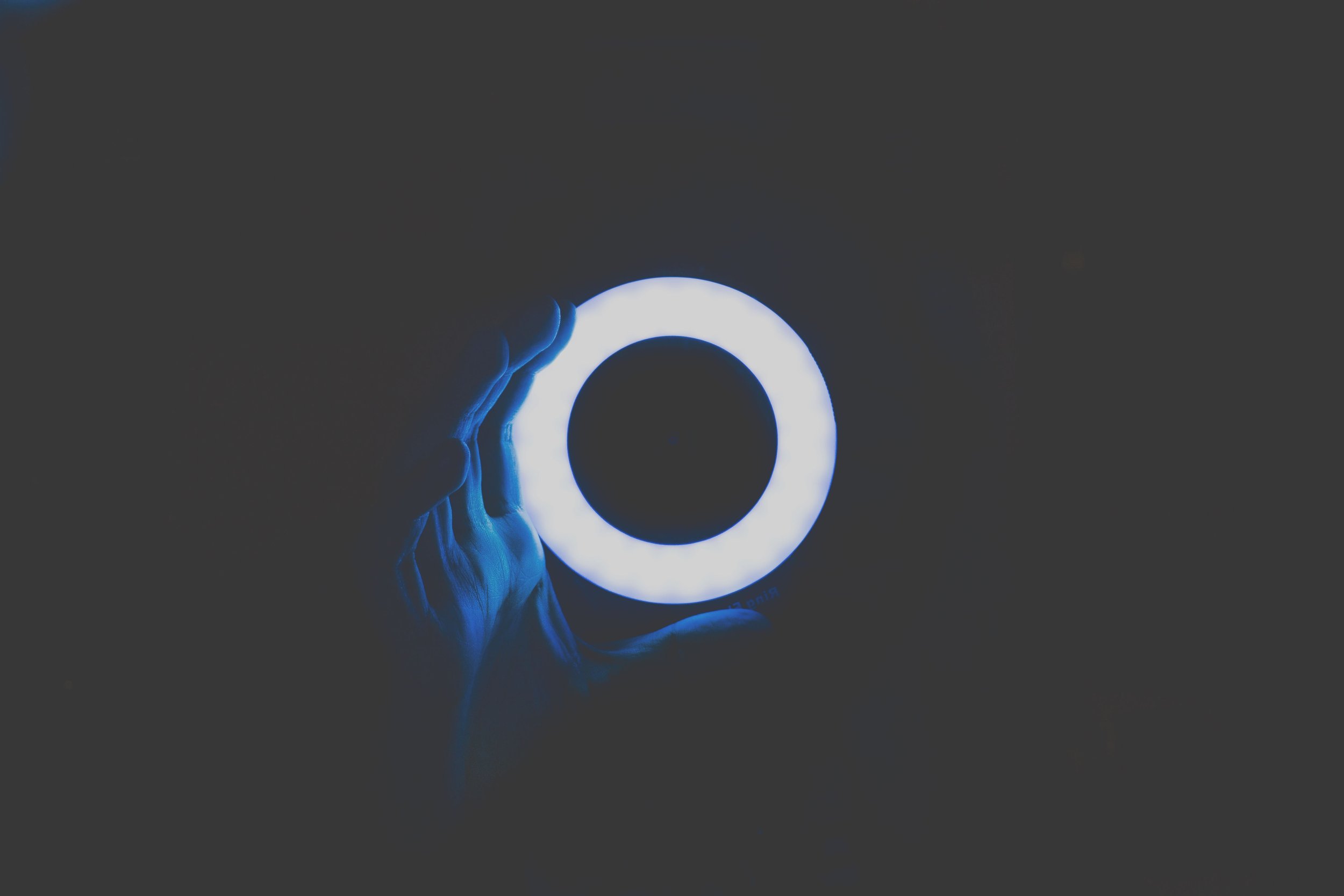
Preventing these types of situations directly impacts the type of algorithm that can be used to assist in decisions affecting individuals because they must be algorithms capable of explaining or arguing why they make a certain recommendation and not another or why they formulate a certain prediction. In fact, there is a relatively new branch of AI that has gained much traction, known as explainable AI, which addresses these issues. And so far, it is difficult to achieve that deep learning methods, which are making very good and very fast predictions about very complex realities, can adequately justify those predictions. This indeed occurs with all black-box algorithms, including not only those of deep learning but also all those based on artificial neural networks or evolutionary computation.
The application ChatGPT from Open AI, released to the general public on November 30, 2022, has a remarkable ability to draft all kinds of texts or lines of code on any topic it is questioned about; the barriers to the universalization of the electric car, drafting a standard rental contract, or the code for an application that makes an avatar wave hello only when there is a human in front of the screen are some of the topics it can provide answers about.
In all the tests conducted by the authors and many other users, the speed and plausibility of the responses offered by the application are surprising, although there are also observed absences of relevant content or arguments in specific fields that make it difficult to fully consider the results as reliable.
ChatGPT has been trained on millions of documents published up until 2021, but its creators have not revealed what documents they are. Millions of people experimenting with the application at this moment would like to know what the "universe" of knowledge used was to be able to interpret the possible biases in the results it offers.
Too Promising to Pass Up
It seems clear that the ability of algorithms to encompass complexity, bring us closer to a desired objectivity, but above all their capacity to generate economies of scale in activities related to knowledge represents an opportunity too attractive for progress to let go of, despite the known risks, and those others that we will discover in the coming years.
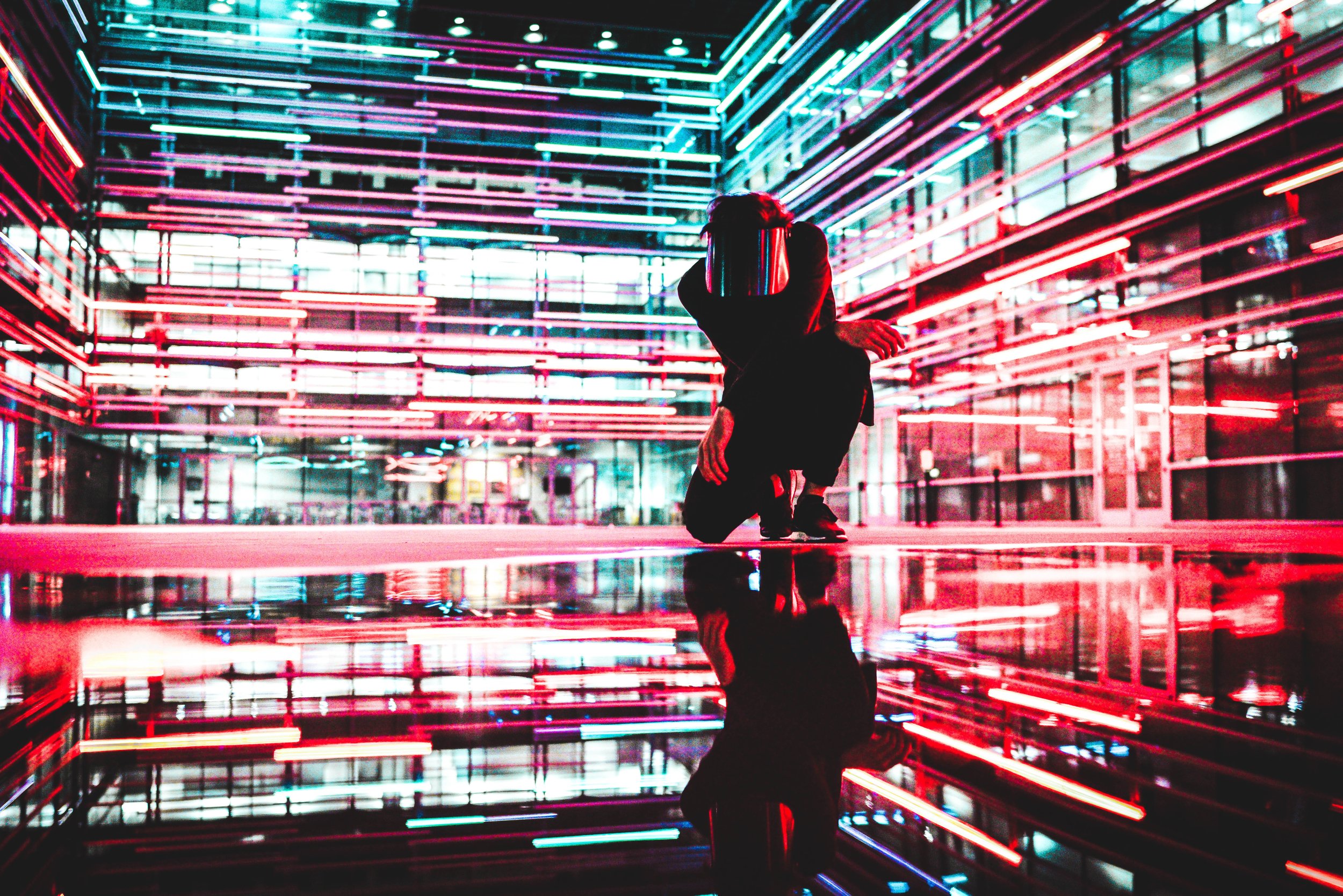
Only through algorithmic organization can the Hong Kong metro efficiently manage the ten thousand workers who each night perform the 2,600 repair and maintenance tasks necessary to provide public transport with extremely high punctuality levels (99.9% since 2014!) and generate savings of 2 days on repairs per week and about $800,000 per year [Chun 2005] [Hodson 2014]. The Barcelona metro has had an AI-based system since December 2020 that allows it to control the passenger flow of platforms and trains, and open or close access to them to create the safest conditions for passengers from the standpoint of virus spread [Nac 2020].
When an algorithm is encoded in a programming language understandable by machines, it becomes a tool that can scale its impact to a dimension unattainable through human-to-human communication. For example, the software update of a fleet of connected vehicles or robots allows incorporating improvements resulting from the group's learning to each of them. In parallel, each update of the algorithms managing our search or navigation tools opens and closes opportunities for businesses and citizens to discover each other.
In reality, we are witnessing the emerging development of a powerful technology, Artificial Intelligence, which, like all new things, generates certain fears and where good information can help dispel doubts. In this sense, the Catalan Artificial Intelligence Strategy [catalonia.ai 2021] launched a public course on Artificial Intelligence in 2021 aimed at providing basic training to the general public. The course, designed by the UPC research center, IDEAI (Intelligent Data Science and Artificial Intelligence research center), is free and can be accessed from the website https://ciutadanIA.cat.
Like all technologies, AI can present more or less ethical uses, with greater or lesser risk, and the current challenge is to find that delimitation in the uses of AI that allows us to take advantage of all of its benefits without exposing us to harm.
If we look back in history, fire or the knife are technologies that, when they appear, drastically change the history of humanity. Both, like AI and many others, have two sides. Fire allowed us to warm up and survive freezing temperatures, and also to cook, but if precautions are not taken, it can cause burns and fires that can lead to major natural disasters. The knife enabled new manipulations of food and the shaping of new tools contributing to the development of civilization, but it also serves to harm people; evidence of this is that in all cultures, we have developed rules that penalize the undesirable uses of these technologies. However, despite these risks, no one thinks to prohibit the manufacture and use of knives to protect us from their dangers and risks. And this is precisely what should also happen with Artificial Intelligence; it is more about identifying risks and regulating its uses to allow for beneficial development for all.
Trust, Machine Limits, and the Power of Data
If machine learning maximizes a function by applying computational power, speed, and the learning capacity of machines to a mass of data, then it is worth evaluating those dimensions in which machines are reliable and those situations in which the data mass is appropriate.
Then the question is: Who can we trust our "mass of data" to? Who can we trust to control the machines to work on our behalf?
The Edelman report 2022 indicates that globally we are at the lowest level of trust in businesses, NGOs, institutions, and the media since they began this series in the year 2000. The succession of financial crises, institutional corruption, fake news, and Covid-19 have installed distrust as the default sentiment towards institutions. The Dutch government as a whole was forced to resign on January 8, 2021, when it could be demonstrated that the SyRI system based on AI that had been used since 2014 to identify fraud among welfare beneficiaries suffered from a bias that only implicated immigrant families from vulnerable districts and had wrongly processed 26,000 families, forcing them to return subsidies unjustly. Hundreds of families suffering this unjust institutional harassment, causing depression, ruin, stigmatization, suicides, or imprisonment BY ERROR, because no one critically reviewed the algorithm's recommendations [Lazcoz 2022].
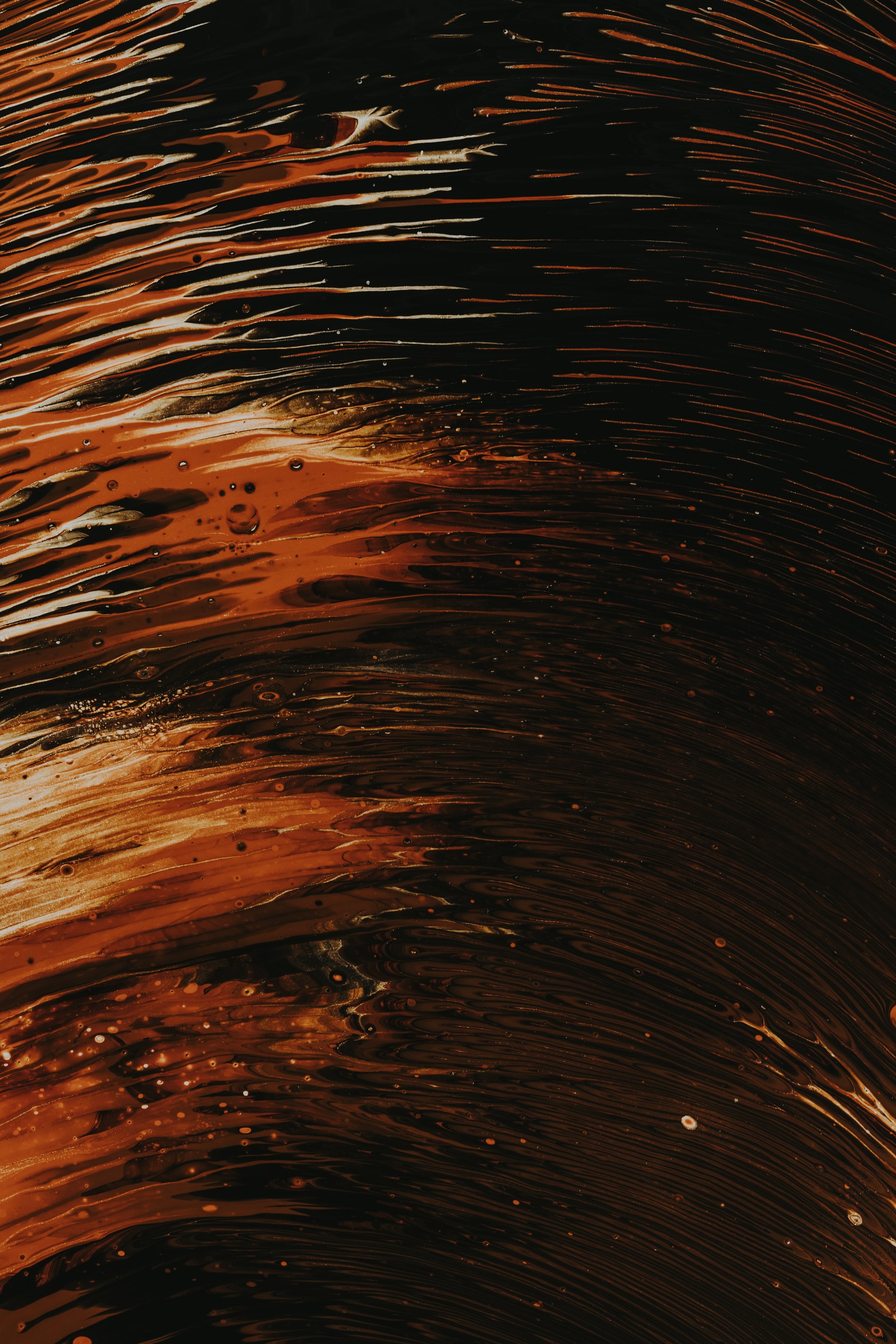
In the digital realm, Tim Berners Lee, the creator of the web, criticizes how his own creation, initially intended to be the greatest tool for knowledge democratization in history, has degenerated into an instrument of division and inequality through attention capture and behavior control and attempts to develop a better alternative.
On the other hand, the development of new technologies has led to a concentration of algorithms and data in the hands of a few global super-corporations that increasingly influence more aspects of our lives, with risks that this entails.
Ensuring that algorithms do not incorporate biases by design is one of the greatest challenges we face. In fact, the design of algorithms relies on the understanding the developer has of the real process being computationally represented, and to acquire this understanding, one must interact with the expert in that process and capture the relevant aspects to consider in the implementation.
In this transmission from the domain expert to the computing specialist, implicit knowledge plays many bad tricks. Neither the domain expert is aware that they have it, nor that they are using it in their reasoning, decisions, and actions, nor do they realize that they are not including it in their world description, nor are they transmitting it to the interlocutor, nor is the developer aware that they make (often dangerously oversimplifying) hypotheses that guide their implementation and can skew the algorithm's behavior.
A good part of implicit knowledge has a situated cultural component; that is, many social values are valid in a specific society or situation but are not universalizable, and some criteria are activated appropriately in humans vis-à-vis certain exceptional situations, but remain in the unconscious most of the time, and therefore, cannot be conveyed either to the verbal nor much less to the algorithm.
Machines can apply computational power, speed, and processing capability, but they are not sensitive to context, unless given a good formal description of it, they are incapable of dealing with exceptions if they have not been implemented to take them into account, nor are they capable of dealing with unforeseen events, unlike humans. This can lead to biased behaviors in algorithms that deal with very complex phenomena.
Such biases are not always intentional. Often we do not practice sufficiently the thorough analysis of the scenarios for which algorithms are built. Defining criteria for the majority, for the general case, is almost always a bad idea because it is in the exception, in the violation of the minority, where injustices appear.
It is necessary to employ more lateral thinking and conduct a more thorough analysis of the potential exceptional scenarios to reduce the bias in the algorithms' logic. Certainly, having diverse teams facilitates this task, as the combination of different perspectives on the same problem brings complementary views that naturally reduce logical biases, but also biases in the data.
On the other hand, algorithms are fed with data, and we have lost the good habit of using the old theory of sampling and experimental design to ensure that the data we use to train an AI will adequately represent the population under study and will not carry biases that will corrupt the predictions and recommendations of the resulting systems.
As explained by Kai Fu Lee in his book "AI Superpowers", the availability of data is more relevant than the algorithm's quality. For example, chess algorithms existed since 1983, but it wasn't until 1997 that Deep Blue defeated Kasparov, just six years after a database with 700,000 games between masters was published. In a world where basic algorithms are public, it is the availability of data that determines the advantage.
However, currently, data no longer only refers to numbers or measurable quantities that traditional statistics has been analyzing for so many years. Voice, images, videos, documents, tweets, opinions, our vital signs or values of virtual currencies are today invaluable sources of information from which to extract relevant information in all directions and constitute a new generation of complex data that are extensively exploited from the realm of artificial intelligence. Video games, virtual simulations, or the much-anticipated metaverse are all built with data and represent computational metaphors of real or imagined worlds, and as Beau Cronin states, they may be preferable to the real world for those who do not enjoy the "privilege of reality": it is striking to note that nowadays 50% of young people already believe they have more life opportunities in the online environment than in the real world.
Decentralized or distributed data architectures, along with the emerging technologies of federated data science, are part of an intense debate about how to develop data policies, which not only address the support architectures for storing data but also how they are produced, policies for data openness and ownership models (commercial or institutional?) and use licenses. Some argue that if the production of data is distributed - the facial recognition algorithm "facelift" from Google is based on photographs of 82,000 people - its ownership should also be distributed. In some cases, the courts have also mandated the destruction of algorithms generated through the misleading acquisition of data, like the Kurbo application that captured data from eight-year-old children without their guardians' knowledge.
Challenges and Proposals
There is no longer any doubt that human activity modifies living conditions on the planet and that it has done so at an accelerated pace over the last two centuries, to the point of making us aware of the climate and social emergency we live in.
Our collective challenge now is life: how to generate conditions for a decent life for the 8 billion people inhabiting the planet, and how to do so without our survival threatening the quantity, quality, and diversity of life of other living beings or future generations.
We cannot do without Artificial Intelligence as a tool to encompass the complexity, simultaneity, and scale of these challenges, but as we have argued earlier, we also cannot let that which is technically possible, although not necessarily desirable, guide us in its development.
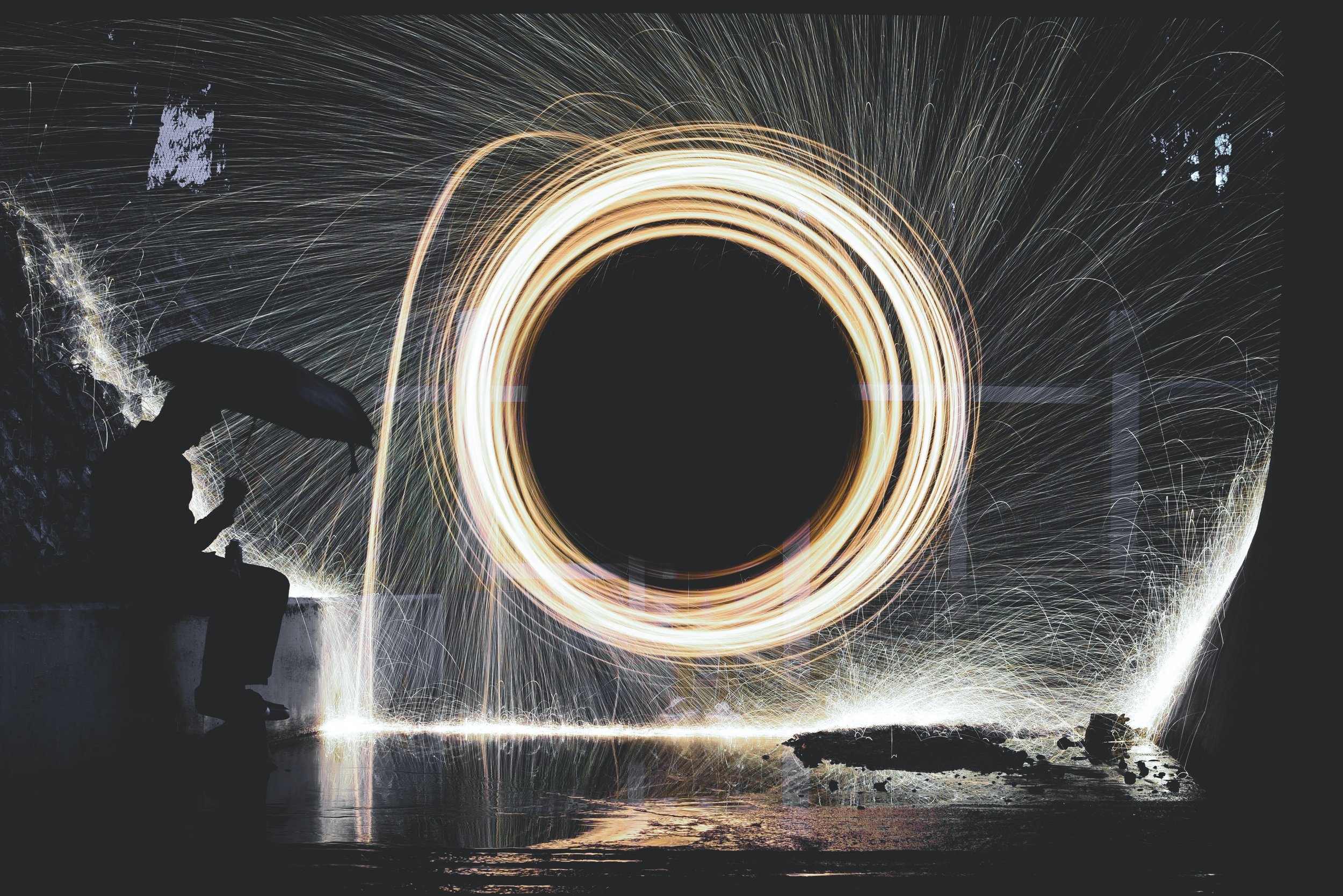
Only through algorithmic organization can the Hong Kong metro efficiently manage the ten thousand workers who each night perform the 2,600 repair and maintenance tasks necessary to provide public transport with extremely high punctuality levels (99.9% since 2014!) and generate savings of 2 days on repairs per week and about $800,000 per year [Chun 2005] [Hodson 2014]. The Barcelona metro has had an AI-based system since December 2020 that allows it to control the passenger flow of platforms and trains, and open or close access to them to create the safest conditions for passengers from the standpoint of virus spread [Nac 2020].
When an algorithm is encoded in a programming language understandable by machines, it becomes a tool that can scale its impact to a dimension unattainable through human-to-human communication. For example, the software update of a fleet of connected vehicles or robots allows incorporating improvements resulting from the group's learning to each of them. In parallel, each update of the algorithms managing our search or navigation tools opens and closes opportunities for businesses and citizens to discover each other.
In reality, we are witnessing the emerging development of a powerful technology, Artificial Intelligence, which, like all new things, generates certain fears and where good information can help dispel doubts. In this sense, the Catalan Artificial Intelligence Strategy [catalonia.ai 2021] launched a public course on Artificial Intelligence in 2021 aimed at providing basic training to the general public. The course, designed by the UPC research center, IDEAI (Intelligent Data Science and Artificial Intelligence research center), is free and can be accessed from the website https://ciutadanIA.cat.
Like all technologies, AI can present more or less ethical uses, with greater or lesser risk, and the current challenge is to find that delimitation in the uses of AI that allows us to take advantage of all its benefits without exposing us to harm.
If we look back in history, fire or the knife are technologies that, when they appear, drastically change the history of humanity. Both, like AI and many others, have two sides. Fire allowed us to warm up and survive freezing temperatures, and also to cook, but if precautions are not taken, it can cause burns and fires that can lead to major natural disasters. The knife enabled new manipulations of food and the shaping of new tools contributing to the development of civilization, but it also serves to harm people; evidence of this is that in all cultures, we have developed rules that penalize the undesirable uses of these technologies. However, despite these risks, no one thinks to prohibit the manufacture and use of knives to protect us from their dangers and risks. And this is precisely what should also happen with Artificial Intelligence; it is more about identifying risks and regulating its uses to allow for beneficial development for all.
Trust, Machine Limits, and the Power of Data
If machine learning maximizes a function by applying computational power, speed, and the learning capacity of machines to a mass of data, then it is worth evaluating those dimensions in which machines are reliable and those situations in which the data mass is appropriate.
Then the question is: Who can we trust our "mass of data" to? Who can we trust to control the machines to work on our behalf?
The Edelman report 2022 indicates that globally we are at the lowest level of trust in businesses, NGOs, institutions, and the media since they began this series in the year 2000. The succession of financial crises, institutional corruption, fake news, and Covid-19 have installed distrust as the default sentiment toward institutions. The Dutch government as a whole was forced to resign on January 8, 2021, when it could be demonstrated that the SyRI system based on AI that had been used since 2014 to identify fraud among welfare beneficiaries suffered from a bias that only implicated immigrant families from vulnerable districts and had wrongly processed 26,000 families, forcing them to return subsidies unjustly. Hundreds of families suffering this unjust institutional harassment, causing depression, ruin, stigmatization, suicides, or imprisonment BY ERROR, because no one critically reviewed the algorithm's recommendations [Lazcoz 2022].
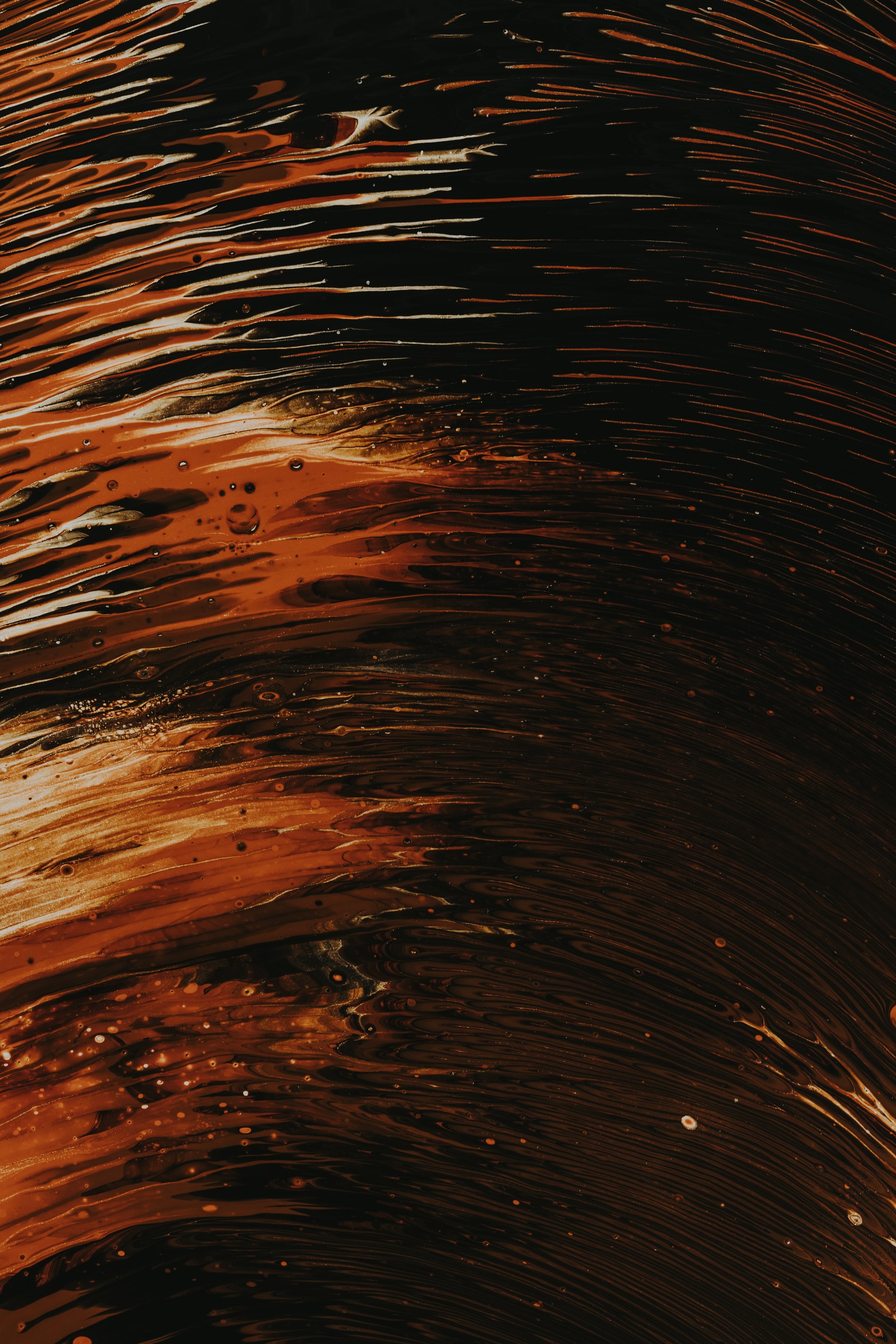
In the digital realm, Tim Berners Lee, the creator of the web, criticizes how his own creation, initially intended to be the greatest tool for knowledge democratization in history, has degenerated into an instrument of division and inequality through attention capture and behavior control and attempts to develop a better alternative.
On the other hand, the development of new technologies has led to a concentration of algorithms and data in the hands of a few global super-corporations that increasingly influence more aspects of our lives, with risks that this entails.
Ensuring that algorithms do not incorporate biases by design is one of the greatest challenges we face. In fact, the design of algorithms relies on the understanding the developer has of the real process being computationally represented, and to acquire this understanding, one must interact with the expert in that process and capture the relevant aspects to consider in the implementation.
In this transmission from the domain expert to the computing specialist, implicit knowledge plays many bad tricks. Neither the domain expert is aware that they have it, nor that they are using it in their reasoning, decisions, and actions, nor do they realize that they are not including it in their world description, nor are they transmitting it to the interlocutor, nor is the developer aware that they make (often dangerously oversimplifying) hypotheses that guide their implementation and can skew the algorithm's behavior.
A good part of implicit knowledge has a situated cultural component; that is, many social values are valid in a specific society or situation but are not universalizable, and some criteria are activated appropriately in humans vis-à-vis certain exceptional situations, but remain in the unconscious most of the time, and therefore, cannot be conveyed either to the verbal nor much less to the algorithm.
Machines can apply computational power, speed, and processing capability, but they are not sensitive to context, unless given a good formal description of it, they are incapable of dealing with exceptions if they have not been implemented to take them into account, nor are they capable of dealing with unforeseen events, unlike humans. This can lead to biased behaviors in algorithms that deal with very complex phenomena.
Such biases are not always intentional. Often we do not practice sufficiently the thorough analysis of the scenarios for which algorithms are built. Defining criteria for the majority, for the general case, is almost always a bad idea because it is in the exception, in the violation of the minority, where injustices appear.
It is necessary to employ more lateral thinking and conduct a more thorough analysis of the potential exceptional scenarios to reduce the bias in the algorithms' logic. Certainly, having diverse teams facilitates this task, as the combination of different perspectives on the same problem brings complementary views that naturally reduce logical biases, but also biases in the data.
On the other hand, algorithms are fed with data, and we have lost the good habit of using the old theory of sampling and experimental design to ensure that the data we use to train an AI will adequately represent the population under study and will not carry biases that will corrupt the predictions and recommendations of the resulting systems.
As explained by Kai Fu Lee in his book "AI Superpowers", the availability of data is more relevant than the algorithm's quality. For example, chess algorithms existed since 1983, but it wasn't until 1997 that Deep Blue defeated Kasparov, just six years after a database with 700,000 games between masters was published. In a world where basic algorithms are public, it is the availability of data that determines the advantage.
However, currently, data no longer only refers to numbers or measurable quantities that traditional statistics has been analyzing for so many years. Voice, images, videos, documents, tweets, opinions, our vital signs or values of virtual currencies are today invaluable sources of information from which to extract relevant information in all directions and constitute a new generation of complex data that are extensively exploited from the realm of artificial intelligence. Video games, virtual simulations, or the much-anticipated metaverse are all built with data and represent computational metaphors of real or imagined worlds, and as Beau Cronin states, they may be preferable to the real world for those who do not enjoy the "privilege of reality": it is striking to note that nowadays 50% of young people already believe they have more life opportunities in the online environment than in the real world.
Decentralized or distributed data architectures, along with the emerging technologies of federated data science, are part of an intense debate about how to develop data policies, which not only address the support architectures for storing data but also how they are produced, policies for data openness and ownership models (commercial or institutional?) and use licenses. Some argue that if the production of data is distributed - the facial recognition algorithm "facelift" from Google is based on photographs of 82,000 people - its ownership should also be distributed. In some cases, the courts have also mandated the destruction of algorithms generated through the misleading acquisition of data, like the Kurbo application that captured data from eight-year-old children without their guardians' knowledge.
Challenges and Proposals
There is no longer any doubt that human activity modifies living conditions on the planet and that it has done so at an accelerated pace over the last two centuries, to the point of making us aware of the climate and social emergency we live in.
Our collective challenge now is life: how to generate conditions for a decent life for the 8 billion people inhabiting the planet, and how to do so without our survival threatening the quantity, quality, and diversity of life of other living beings or future generations.
We cannot do without Artificial Intelligence as a tool to encompass the complexity, simultaneity, and scale of these challenges, but as we have argued earlier, we also cannot let that which is technically possible, although not necessarily desirable, guide us in its development.
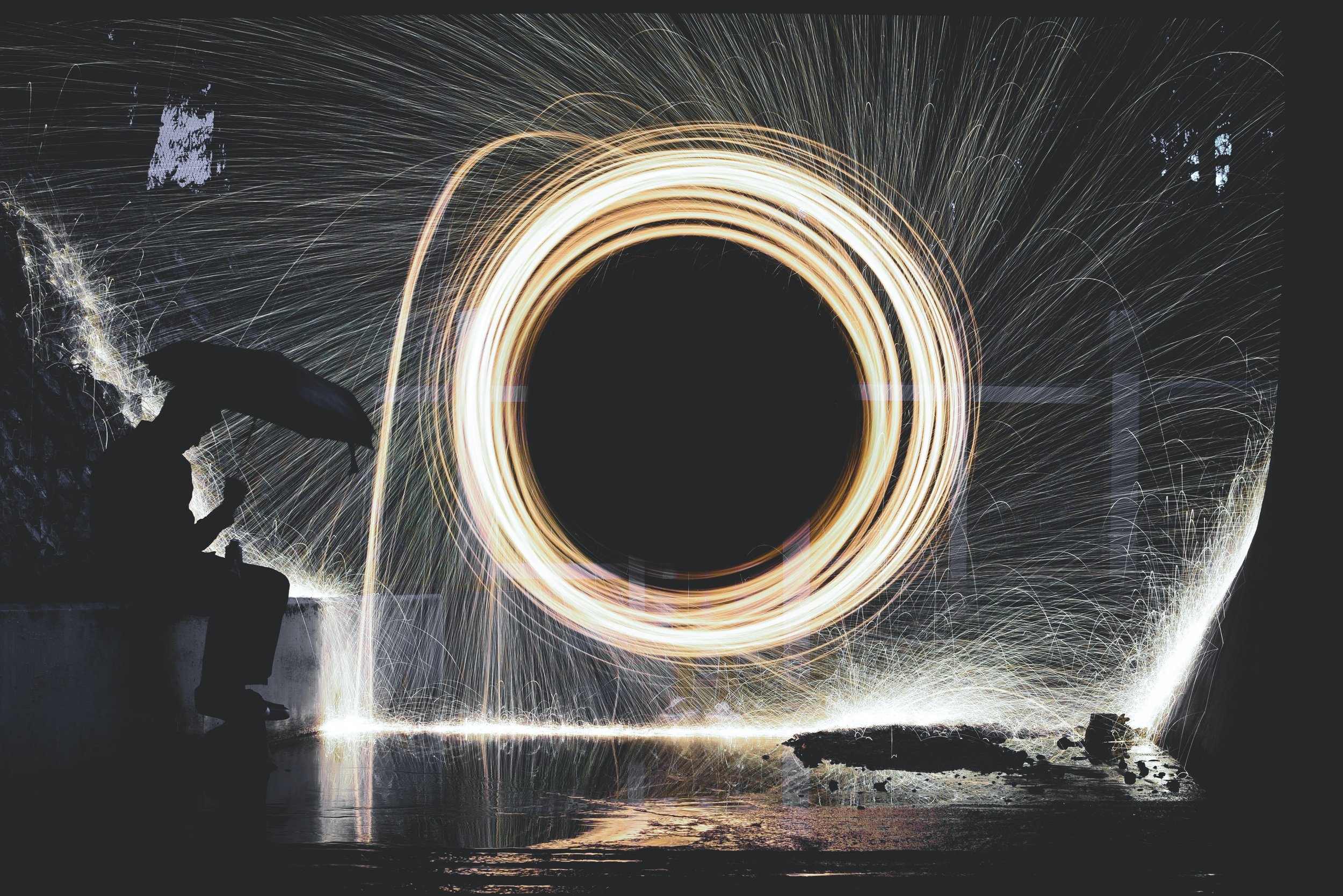
Only through algorithmic organization can the Hong Kong metro efficiently manage the ten thousand workers who each night perform the 2,600 repair and maintenance tasks necessary to provide public transport with extremely high punctuality levels (99.9% since 2014!) and generate savings of 2 days on repairs per week and about $800,000 per year [Chun 2005] [Hodson 2014]. The Barcelona metro has had an AI-based system since December 2020 that allows it to control the passenger flow of platforms and trains, and open or close access to them to create the safest conditions for passengers from the standpoint of virus spread [Nac 2020].
When an algorithm is encoded in a programming language understandable by machines, it becomes a tool that can scale its impact to a dimension unattainable through human-to-human communication. For example, the software update of a fleet of connected vehicles or robots allows incorporating improvements resulting from the group's learning to each of them. In parallel, each update of the algorithms managing our search or navigation tools opens and closes opportunities for businesses and citizens to discover each other.
In reality, we are witnessing the emerging development of a powerful technology, Artificial Intelligence, which, like all new things, generates certain fears and where good information can help dispel doubts. In this sense, the Catalan Artificial Intelligence Strategy [catalonia.ai 2021] launched a public course on Artificial Intelligence in 2021 aimed at providing basic training to the general public. The course, designed by the UPC research center, IDEAI (Intelligent Data Science and Artificial Intelligence research center), is free and can be accessed from the website https://ciutadanIA.cat.
Like all technologies, AI can present more or less ethical uses, with greater or lesser risk, and the current challenge is to find that delimitation in the uses of AI that allows us to take advantage of all its benefits without exposing us to harm.
If we look back in history, fire or the knife are technologies that, when they appear, drastically change the history of humanity. Both, like AI and many others, have two sides. Fire allowed us to warm up and survive freezing temperatures, and also to cook, but if precautions are not taken, it can cause burns and fires that can lead to major natural disasters. The knife enabled new manipulations of food and the shaping of new tools contributing to the development of civilization, but it also serves to harm people; evidence of this is that in all cultures, we have developed rules that penalize the undesirable uses of these technologies. However, despite these risks, no one thinks to prohibit the manufacture and use of knives to protect us from their dangers and risks. And this is precisely what should also happen with Artificial Intelligence; it is more about identifying risks and regulating its uses to allow for beneficial development for all.
Trust, Machine Limits, and the Power of Data
If machine learning maximizes a function by applying computational power, speed, and the learning capacity of machines to a mass of data, then it is worth evaluating those dimensions in which machines are reliable and those situations in which the data mass is appropriate.
Then the question is: Who can we trust our "mass of data" to? Who can we trust to control the machines to work on our behalf?
The Edelman report 2022 indicates that globally we are at the lowest level of trust in businesses, NGOs, institutions, and the media since they began this series in the year 2000. The succession of financial crises, institutional corruption, fake news, and Covid-19 have installed distrust as the default sentiment toward institutions. The Dutch government as a whole was forced to resign on January 8, 2021, when it could be demonstrated that the SyRI system based on AI that had been used since 2014 to identify fraud among welfare beneficiaries suffered from a bias that only implicated immigrant families from vulnerable districts and had wrongly processed 26,000 families, forcing them to return subsidies unjustly. Hundreds of families suffering this unjust institutional harassment, causing depression, ruin, stigmatization, suicides, or imprisonment BY ERROR, because no one critically reviewed the algorithm's recommendations [Lazcoz 2022].
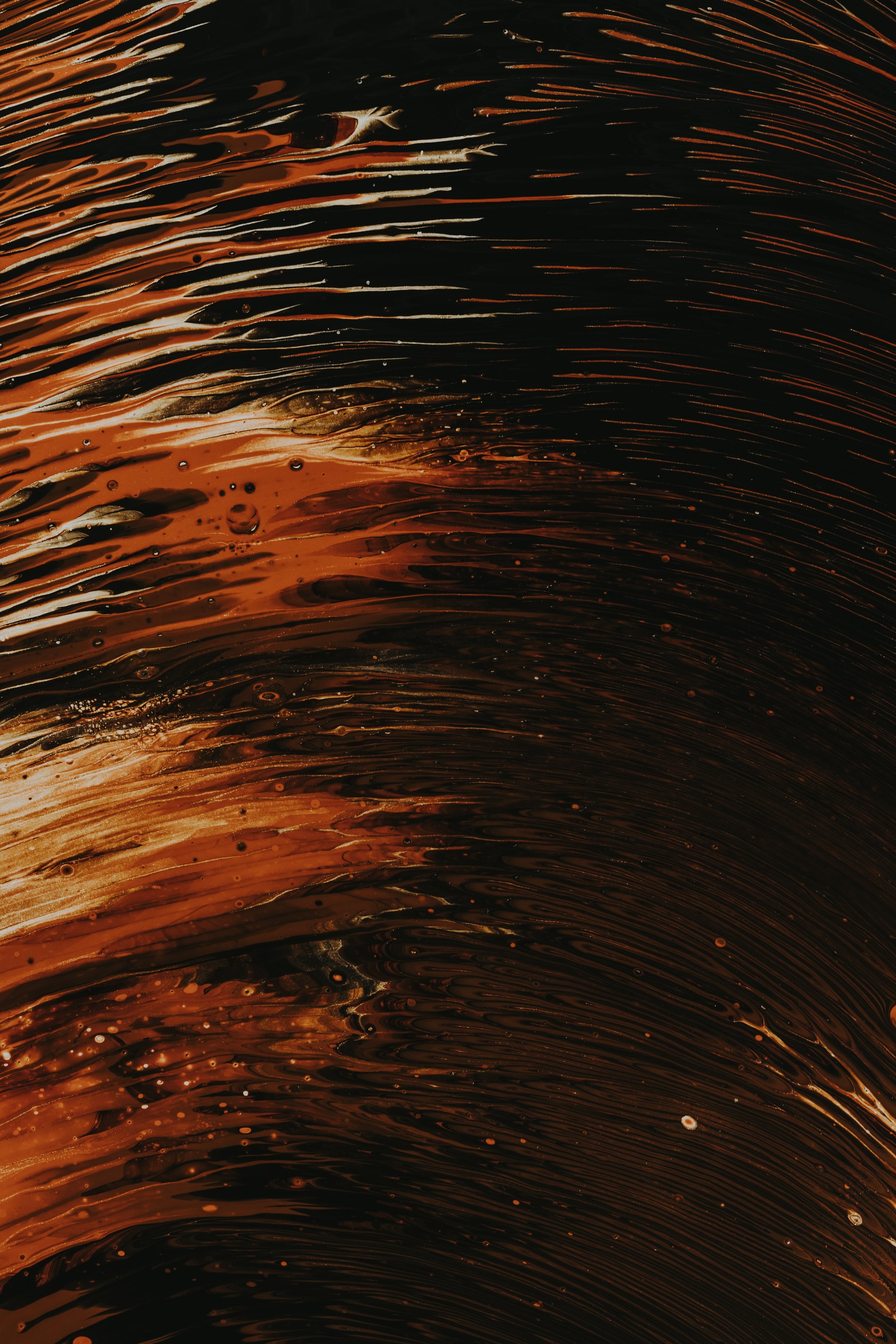
In the digital realm, Tim Berners Lee, the creator of the web, criticizes how his own creation, initially intended to be the greatest tool for knowledge democratization in history, has degenerated into an instrument of division and inequality through attention capture and behavior control and attempts to develop a better alternative.
On the other hand, the development of new technologies has led to a concentration of algorithms and data in the hands of a few global super-corporations that increasingly influence more aspects of our lives, with risks that this entails.
Ensuring that algorithms do not incorporate biases by design is one of the greatest challenges we face. In fact, the design of algorithms relies on the understanding the developer has of the real process being computationally represented, and to acquire this understanding, one must interact with the expert in that process and capture the relevant aspects to consider in the implementation.
In this transmission from the domain expert to the computing specialist, implicit knowledge plays many bad tricks. Neither the domain expert is aware that they have it, nor that they are using it in their reasoning, decisions, and actions, nor do they realize that they are not including it in their world description, nor are they transmitting it to the interlocutor, nor is the developer aware that they make (often dangerously oversimplifying) hypotheses that guide their implementation and can skew the algorithm's behavior.
A good part of implicit knowledge has a situated cultural component; that is, many social values are valid in a specific society or situation but are not universalizable, and some criteria are activated appropriately in humans vis-à-vis certain exceptional situations, but remain in the unconscious most of the time, and therefore, cannot be conveyed either to the verbal nor much less to the algorithm.
Machines can apply computational power, speed, and processing capability, but they are not sensitive to context, unless given a good formal description of it, they are incapable of dealing with exceptions if they have not been implemented to take them into account, nor are they capable of dealing with unforeseen events, unlike humans. This can lead to biased behaviors in algorithms that deal with very complex phenomena.
Such biases are not always intentional. Often we do not practice sufficiently the thorough analysis of the scenarios for which algorithms are built. Defining criteria for the majority, for the general case, is almost always a bad idea because it is in the exception, in the violation of the minority, where injustices appear.
It is necessary to employ more lateral thinking and conduct a more thorough analysis of the potential exceptional scenarios to reduce the bias in the algorithms' logic. Certainly, having diverse teams facilitates this task, as the combination of different perspectives on the same problem brings complementary views that naturally reduce logical biases, but also biases in the data.
On the other hand, algorithms are fed with data, and we have lost the good habit of using the old theory of sampling and experimental design to ensure that the data we use to train an AI will adequately represent the population under study and will not carry biases that will corrupt the predictions and recommendations of the resulting systems.
As explained by Kai Fu Lee in his book "AI Superpowers", the availability of data is more relevant than the algorithm's quality. For example, chess algorithms existed since 1983, but it wasn't until 1997 that Deep Blue defeated Kasparov, just six years after a database with 700,000 games between masters was published. In a world where basic algorithms are public, it is the availability of data that determines the advantage.
However, currently, data no longer only refers to numbers or measurable quantities that traditional statistics has been analyzing for so many years. Voice, images, videos, documents, tweets, opinions, our vital signs or values of virtual currencies are today invaluable sources of information from which to extract relevant information in all directions and constitute a new generation of complex data that are extensively exploited from the realm of artificial intelligence. Video games, virtual simulations, or the much-anticipated metaverse are all built with data and represent computational metaphors of real or imagined worlds, and as Beau Cronin states, they may be preferable to the real world for those who do not enjoy the "privilege of reality": it is striking to note that nowadays 50% of young people already believe they have more life opportunities in the online environment than in the real world.
Decentralized or distributed data architectures, along with the emerging technologies of federated data science, are part of an intense debate about how to develop data policies, which not only address the support architectures for storing data but also how they are produced, policies for data openness and ownership models (commercial or institutional?) and use licenses. Some argue that if the production of data is distributed - the facial recognition algorithm "facelift" from Google is based on photographs of 82,000 people - its ownership should also be distributed. In some cases, the courts have also mandated the destruction of algorithms generated through the misleading acquisition of data, like the Kurbo application that captured data from eight-year-old children without their guardians' knowledge.
Challenges and Proposals
There is no longer any doubt that human activity modifies living conditions on the planet and that it has done so at an accelerated pace over the last two centuries, to the point of making us aware of the climate and social emergency we live in.
Our collective challenge now is life: how to generate conditions for a decent life for the 8 billion people inhabiting the planet, and how to do so without our survival threatening the quantity, quality, and diversity of life of other living beings or future generations.
We cannot do without Artificial Intelligence as a tool to encompass the complexity, simultaneity, and scale of these challenges, but as we have argued earlier, we also cannot let that which is technically possible, although not necessarily desirable, guide us in its development.
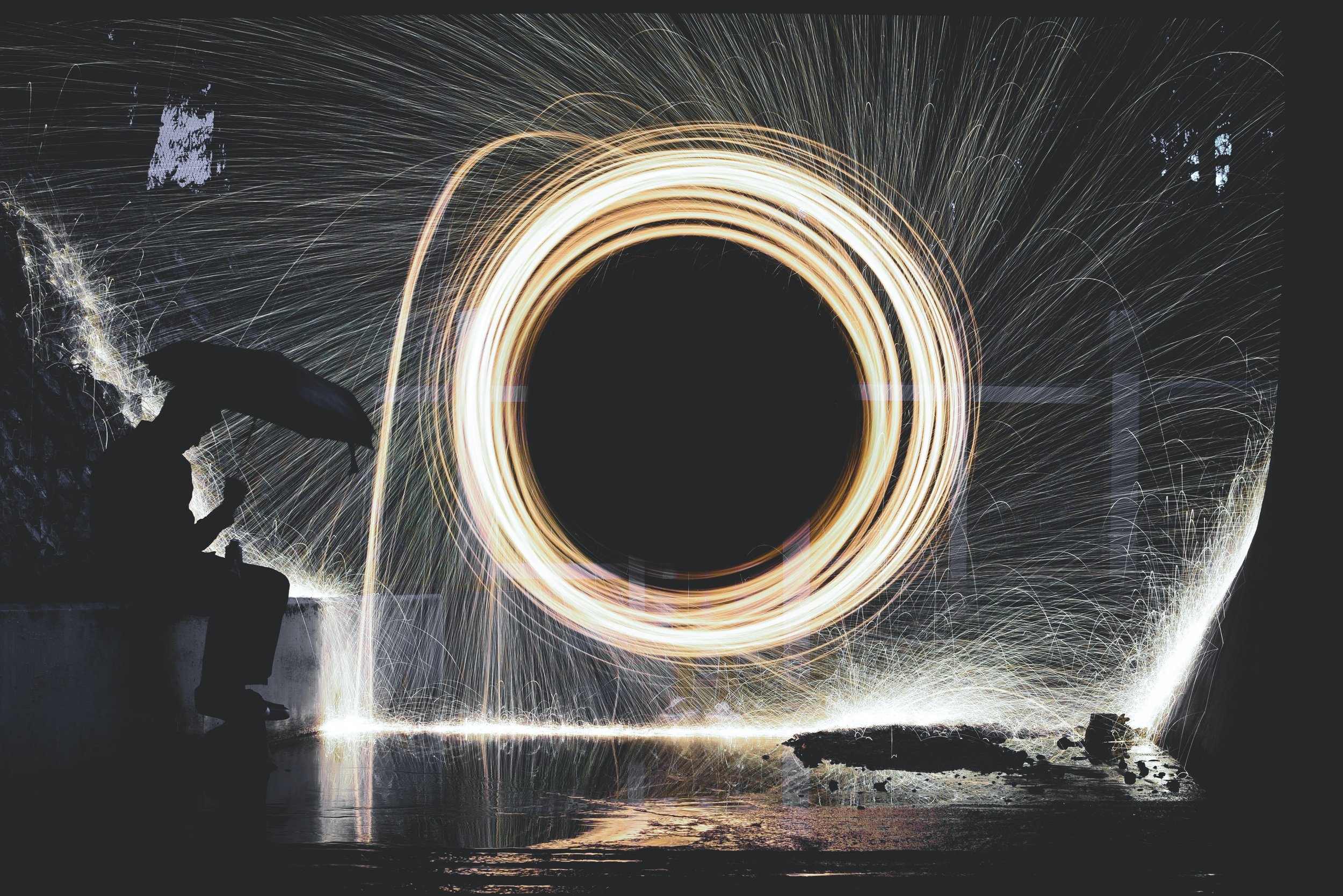